Welcome to the Data Score newsletter, composed by DataChorus LLC. The newsletter is your go-to source for insights into the world of data-driven decision-making. Whether you're an insight seeker, a unique data company, a software-as-a-service provider, or an investor, this newsletter is for you. I'm Jason DeRise, a seasoned expert in the field of data-driven insights. As one of the first 10 members of UBS Evidence Lab, I was at the forefront of pioneering new ways to generate actionable insights from alternative data. Before that, I successfully built a sell-side equity research franchise based on proprietary data and non-consensus insights. After moving on from UBS Evidence Lab, I’ve remained active in the intersection of data, technology, and financial insights. Through my extensive experience as a purchaser and creator of data, I have gained a unique perspective, which I am sharing through the newsletter.
On May 3, 2023, The Data Score Newsletter published one of the more popular entries, sharing my approach to vetting data vendors, which includes eight areas of evaluation. Many of my contacts at data companies reached out to share with me that they hadn’t realized the process to assess data sources was so involved. Meanwhile, my financial market contacts who are buyers of data shared with me that the approach outlined is very similar to what they do.
Use a completed Due Diligence Questionnaire (DDQ) to understand the compliance and risk associated with a dataset.
Assess the return on investment (ROI1) by considering how many decisions can be influenced and the potential limitations of the data.
Conduct common-sense, first-principles tests to ensure the data behaves as expected and reflects known events and expected seasonality. It’s surprising how often these types of tests are failed.
Perform back-testing against benchmarks to measure the dataset's correlation with a known KPI2 while avoiding common statistical mistakes that lead to incorrect conclusions.
Assess the transparency of the methodology used for harvesting, cleansing, and enriching the data while respecting proprietary trade secrets.
Evaluate how the data vendor handles feedback and whether they have the capacity for custom work, understanding the potential implications for competitive advantage.
Understand the vendor's competitive set by asking about their closest competitors and their target customer base.
Examine the Service Level Agreement (SLA) for post-delivery service, including response times for errors, communication of code-breaking changes, and availability of sales engineering support.
In this entry of the Data Score Newsletter, let’s dig into one of the eight points of evaluation: Assess the return on investment (ROI) by considering how many decisions can be influenced and the potential limitations of the data.
Understand the cost of the data investment
Raw data is a cost; insights have value. The conversion of raw data to insight-ready data is where investments must be made to generate value. The breadth and impact of the data-driven insights create a return on data investment.
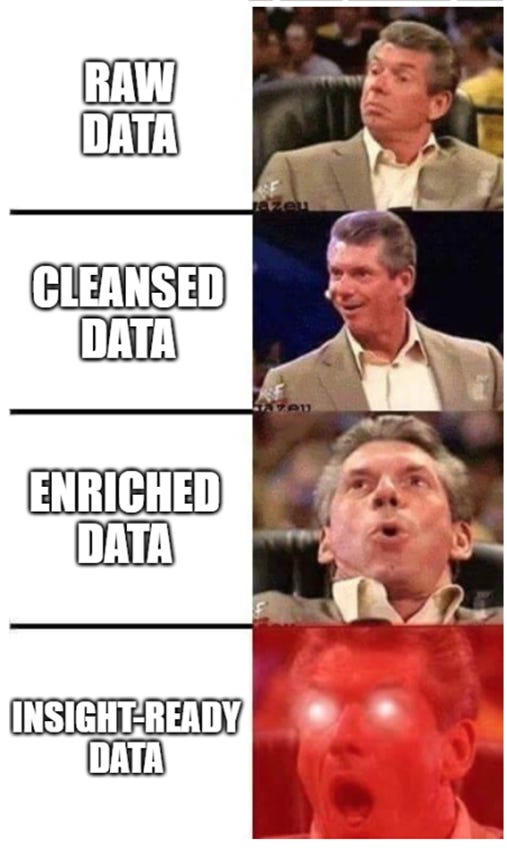
Raw data is an input cost
Raw data on its own has no value. There is still a lingering expectation among professionals that by simply ingesting the most data possible, the answers will easily present themselves, and the decision-makers leveraging the data will have a competitive advantage.