Data Deep Dive: Google Trends, Part I
Google search data is widely accessible, but few understand how to use it correctly or the rigor required to ensure its reliability.
Welcome to the Data Score newsletter, composed by DataChorus LLC. The newsletter is your go-to source for insights into the world of data-driven decision-making. Whether you're an insight seeker, a unique data company, a software-as-a-service provider, or an investor, this newsletter is for you. I'm Jason DeRise, a seasoned expert in the field of data-driven insights. As one of the first 10 members of UBS Evidence Lab, I was at the forefront of pioneering new ways to generate actionable insights from alternative data. Before that, I successfully built a sell-side equity research franchise based on proprietary data and non-consensus insights. After moving on from UBS Evidence Lab, I’ve remained active in the intersection of data, technology, and financial insights. Through my extensive experience as a purchaser and creator of data, I have gained a unique perspective, which I am sharing through the newsletter.
Google Trends is often used as an Alternative Data1 source. But I get frustrated when I see Google Trends misused to support arguments. The data itself isn’t flawed; rather, it’s the misunderstanding of the data that leads to misinterpretation and poor application.
This is an article I have wanted to publish for about a year. The biggest challenge I faced in creating the final version was avoiding going down the rabbit hole that is Google Trends (each returned search data may spark another idea and another search to investigate). The second challenge was picking examples of Google Trends use that are relevant for readers of the Data Score, but without turning it into a full investment thesis (this isn’t investment research). And the final challenge was avoiding writing about anything that would conflict with my day job.
Rick Beato, a well-known YouTuber in the music industry, recently used Google Trends to argue that music is not as good as it used to be and that music is becoming less popular—yes, he does admit he sounds like an old man yelling at clouds. Here’s the part of the video where he uses Google Trends data.
I appreciate what he was trying to say, but he used Google Trends incorrectly. I thought this question of the popularity of music compared to other activities provided a great low-key topic to explore via Google Trends, using the best practices for getting quality results. It also helps contrast good and poor uses of Google Trends.
In the Data Deep Dive Series of The Data Score, the format covers the following topics:
Common questions addressed with the data
Underlying Data
Cleaning the Data
Enriching the data
Limitations to consider
Action items to begin using the data
I went deep into the underlying data in this part of the deep dive. We’ll approach the remaining topics in Part II of the Google Trends deep dive.
Sponsored Advertisement
Exabel published their latest US Consumer Sector Report as of July 2024, which includes data from Facteus, Flywheel Alternative Data, Revelio and newly added Apptopia, Datos, and Placer.ai. The combination of transaction data, web-scraped pricing and demand data, clickstream data, foot traffic data, and app usage data provides multiple angles on the health of the US consumer sector, covering 80+ companies. You can sign up using the link below to receive monthly updates. https://www.exabel.com/us-consumer-sector-report/
Common questions addressed with the data
As a starting point, we need to understand what Google search is and isn’t. It’s not a proxy for purchases. It’s not even a proxy for intent. Google search data serves as a proxy for “top-of-mind awareness,” which refers to the topics or brands that are most immediate in a person's thoughts. When someone uses Google, what they type into the empty search bar reflects what's currently occupying their mind, whether it's curiosity, concern, or intent.
That could be to find a product to purchase or a video they want to watch. Or, it could be that the search term entered is because they want to know more about something that has negative implications for the searcher. For example, a major controversy affecting a brand or product leads to higher search activity. That’s not a positive signal for purchase intent.
For instance, the surge in searches for Delta Airlines during July 21–27, 2024, wasn't indicative of increased purchases (the Crowdstrike/Micrsoft outage affected Delta Airlines materially), just as the spike for Southwest Airlines in December 2022 wasn’t a good signal either (bad weather caused a significant number of Southwest flights to be cancelled).
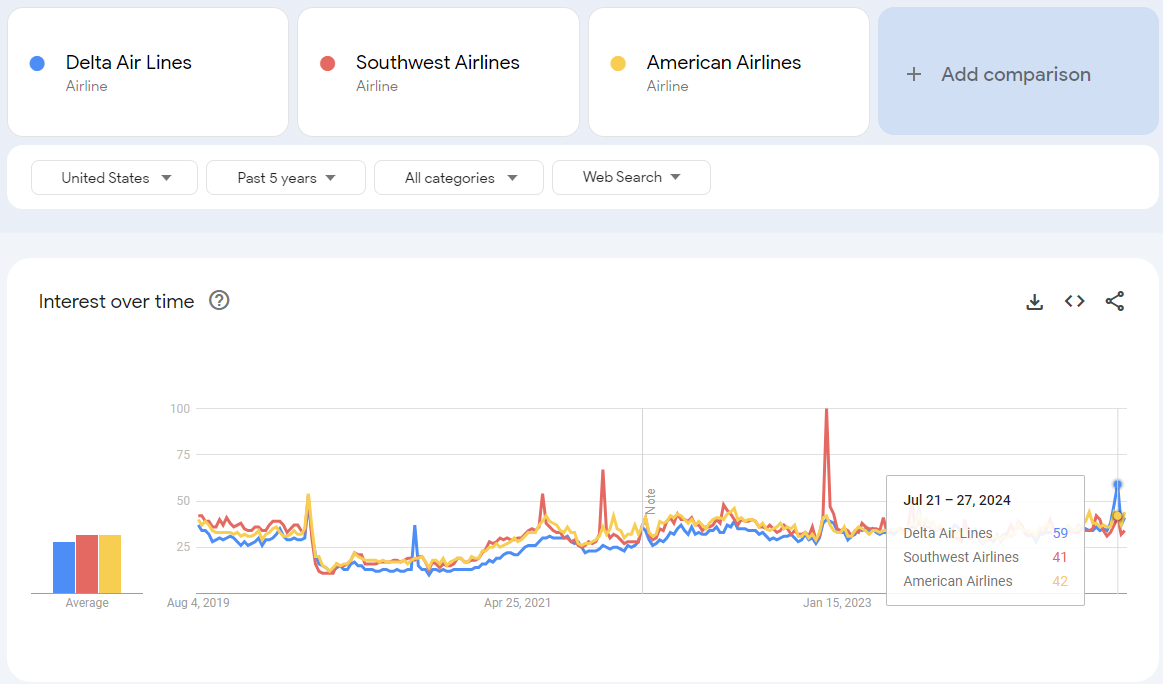
Investment questions that can be answered:
The types of questions that can be answered cover most sectors and geographies. The primary use is to address investment debates, where knowing top-of-mind awareness of a topic would be critical to assessing the potential for outcomes. This could be any topic, primarily with a consumer lens, but is possible for B2B product or service awareness. When does top-of-mind awareness matter the most?
Assess if a disruptive innovation is gaining mindshare
Assess if new consumer behaviors are long-term trend or fad by monitoring awareness
Assess potential success of a new product launch compared to prior product launches based on initial search activity
Assess if the latest media release is likely to be a blockbuster
Assess the rising public consciousness of political topics and themes
Keep in mind each of these questions is an example where there is little known or measurable information about the downstream impacts of changing top-of-mind awareness. Top-of-mind awareness refers to how prominently a topic or brand is in people's thoughts, which is the first step in the marketing funnel toward trial and repeat purchases. High top-of-mind awareness can eventually influence consumer behaviors that impact a company’s sales and profitability, reflected in the company’s revenues and profits. If there are known measures available that capture behaviors further down that marketing funnel, the importance of the questions Google Trends can address as a data point becomes less important (e.g., if we care about the current revenue trend of a retailer, transaction data is a closer proxy). However, combining top-of-mind awareness with a dataset covering purchase or usage behavior allows for a deeper understanding of the conversion of awareness to purchase and usage.
Underlying Data
How does the Google Trends tool work?
Google Trends lets users input any term and see sample data on the search activity for the term relative to all searches within a selected time frame and geography. Google Trends indexes the data so that the search term with the highest activity during the selected time period is set to 100, with all other values scaled relative to that peak. This means the chart shows how each term compares to the most searched term during that time frame. Low search activity or no search activity is shown as 0. However, the tool only limits the number of search terms paired with a geography at a time to a maximum of five.
While it appears straightforward, there are numerous nuances to consider, and it's easy to misinterpret the data if not extracted correctly. The most important aspects to consider are:
The underlying data:
Creating a well-crafted search term—the core of getting the right underlying data
Staying within the page view limits
Cleaning the data, which requires adjustments to how the data is collected (and will be covered in part 2)
Working with the random sample returned
Working with the constraint of 5 searches per extraction
Working with multiple time periods and granularity
Underlying references to how Google Trends works:
https://support.google.com/trends/answer/4365533?hl=en
https://medium.com/google-news-lab/what-is-google-trends-data-and-what-does-it-mean-b48f07342ee8
Creating well-crafted search terms:
The quality of the search terms entered is the most important consideration when creating a Google Trends analysis. The most effective search terms reverse-engineer that critical moment when a user, with limitless options, decides what to search for. They also consider how Google Trends data works. Here are many factors that need to be considered:
Considerations about how terms are interpreted by Google Trends:
One search word covers multiple word searches: For example, if we take Rick Beato’s example of tracking the popularity of music, the search term “music” would include searches for “pop music” and “rock music.”. A search for “Pop Music” as a single search term would return data for searches that include both terms. So consider a venn diagram where the circles of “pop” and “music” overlap and what’s returned is just the overlap.
Implications of Synonyms: Google Trends would provide the search index for the term provided. It does not intuitively consider synonyms. The term “music” would return search data, including searches for “punk rock” or “hip hop.” It would not include searches for the words “rock” or "classical.” We need to think about what people actually search for and why they search. This is where things get tricky.
If we care about all music types and think about how people actually search for information about music, people likely enter much more precise terms than typing in “music.” If I want to know when the new Beabadobee album drops, then I’m typing in “Beabadobee” into that Google Search bar (August 16th, by the way). If I want to know when Muse is going on tour next, I’m typing “Muse Tour” (no signs of a new tour, FYI). I’m definitely not going to find that specific information if I type in "music.” even though my search was about music.
Combining search terms: Use "+" to adjust search terms. “Hip Hop” music could be searched as “Hiphop” or “hip-hop.” And rap music is a subgenere of hip hop, so we would need to combine terms together to create a search term as “hip hop + hiphop +hip-hop + hip-hop + rap.”
Exclude related search terms: Use “-” to adjust search terms; minus works less intuitively than the + sign. If we wanted to create a search term for “pop music,” we would want to use the word “pop” to capture all the potential searches like “pop music,” “pop song,” and “pop hits.” But this would also include searches for “pop up” and “pop it” and “pop culture” and “pop tarts” and “cake pop.” So we would need to use “-” between words to remove all the non-music references to “pop”. So the search term might be something like “pop -up -it -culture -tart -tarts -cake”. Another example is that the search for “Hip hop” could be done through subtraction. We could be start with “hip” and remove references unrelated to music: “hip -pain -replacement -surgery -exercise -flexor -joint.” Note: I’ve had bad luck creating useful terms when the number of exclusives is greater than 2. The reductions start to behave less intuitively than I would expect. So be careful when removing terms.
When crafting search strings, geographic differences need to be considered:
When choosing geographies, consider how prevalent Google is as the search engine of choice. For instance, while Google Trends provides data for China, it's unlikely to represent the majority of the population there.
Selecting the worldwide option is not going to give a global population-weighted answer; its going to give a global search-weighted answer reflecting the mix of Google’s search business globally.
Consider that each geography will return the share of search activity for the country. The values are indexed to 100 for the maximum value. Let’s use the example of the search term “beer".” You can see higher search interest in Canada compared to the US. But the value of 70 in Canada and 66 for the same period in the US doesn't mean the term is searched 6% more frequently in Canada. Instead, it reflects the relative share of searches within each country, meaning the term is proportionally more popular in Canada when considering its smaller population size. The search activity shown by Google Trends is a share of the search, which means the term in Canada relative to all other terms in Canada has a higher share of search activity than in the US (the index is like a market share index, not an absolute value index).
Source: Google Trends: https://trends.google.com/trends/explore?date=all,all&geo=US,CA&q=beer,beer&hl=en We need to make sure the same search term string is used for every geography, which means we need to consider language differences in the same search string.
We also need to consider differences in language when crafting the terms.
Homonyms: Words that are spelled the same but have different meanings present a unique challenge in Google Trends. Even with the removal of all the unwanted “pop” references, we can’t actually remove homonyms. Removing homonyms like “pop” is not possible, as the single-word search term could include references to both “pop music” and “soda” (if you are like my friends from upstate NY). Careful term selection is key to accurate data interpretation.
More practical limitations happen with brands like “Coach” because of all the homonyms of coach (a manager in sports, the seat class on an airplane, a public bus). When a single word has multiple meanings, subtracting additional words from the search cannot remove the potential different intentions of the single word search in Google.
Translation will have an impact on search results.
For example, when creating a search for terms that could be stated in different languages, we need to translate for each. If one wanted to track searches related to finding unemployment benefits across many geographies over time, you may want to create the term “unemployment + desempleo + desempleado + Arbeitslosengeld + Arbeitslosigkeit…” These Spanish and German examples consider that the words “unemployment” and “unemployment benefits” are different in each language (whereas in English, the single word “unemployment” would include searches for “unemployment benefits”).
Homonyms can exist in different languages. For example, if we wanted to track top of mind for business intelligence software, Tableau would be a perfectly fine search term in most geographies except in France and Canada, where Tableau means “table” in French and consumer searches for “table” would be returned along with some searches for the business intelligence software.
In languages with different characters, like Japanese or Chinese, it’s important to know how products are typed. For the iPhone, in most countries, “iPhone” as a term would cover all variants, like “iPhone 14” and “iPhone 15,” noting the space between the iPhone and the variant. But in some languages, the characters combine, like "iPhone14,” which the Google trend term would consider differently than "iphone.” The terms would need to be combined with + for each variant. As I was looking for examples of this in real life, it seems like it’s just written out on the “iPhone 15” on the Apple website in China and Japan. So maybe not the best example anymore if users of Google in Japan search for the new iPhone versions and have a space in their search between “iPhone” and the number (I’m pretty sure years ago we figured out that we needed to treat it differently, but maybe not for the iPhone example).
Misspellings: It’s important to account for common misspellings by combining them into a single search term, such as 'Caribbean + Carribean + Caribean.'
How do you know if a term is generating the right results?
Considering all the potential “gotchas” in the term creation, it’s important to have a reliable reference point to compare the results, ensuring they make logical sense. Below are some ways of sanity checking the returned results.
Intuitive rank order reference points: does the search terms come back in a logical order? If not, maybe something is off in the term creation (or maybe you uncovered a surprising insight)
Other sources may help provide a sanity check: This could include company commentary on an earnings conference call or other types of data like clickstream2 or transaction data. Of course, Google Trend’s top-of-mind awareness measurement can be different from that of those other sources. And we want to show those differences by tracking search activity.
Seasonality: A well-crafted search term should align with expected seasonal trends, such as a spike in US retailer searches around Black Friday.
Event spikes: Look for significant past events, such as major news stories or high-profile product releases. If the search term is accurate, it should show a spike during the exact day or week of the event.
Are homonyms and synonyms affecting the returned search results? By looking at the related terms provided by Google Trends, you can check for rising and top terms associated with the search to assess if any adjustments are needed to the crafted search term. For example, after removing various terms from “pop” to get to just “pop music,” I can see there are more search terms to remove. There are references to Funko toys. Also, there’s a popular musician named Pop Smoke (so now I would need to decide if that counts as “pop music” to keep in my crafted search term or not).
Source: Google Trends search for “pop -up -it -culture -tart -tarts -cake”: https://trends.google.com/trends/explore?date=all&geo=US&q=pop%20-up%20-it%20-culture%20-tart%20-tarts%20-cake&hl=en And then there’s more pages of related topics and queries, which also reveals more terms to remove (“brain,” “ups,” “art,” “pimple,” “that,” “iggy,” “warner”). Eventually you get to the point where there’s too many words to remove “pop -up -it -culture -tart -tarts -cake -funko -out -ups -brain -art -pimple -smoke -that -iggy -warner” and you get an error.
Pre-built terms: I strongly recommend that users of Google Trends create their own terms, but as you type the terms into Google Trends, you will see pop-up options to select a predefined term. If your manually created term resembles the prebuilt term, you’re probably in a good spot for the term created.
Why do I not recommend using the pre-built terms?
You may have noticed that in the image I used earlier from Google Trends related to airlines, I used the pre-built terms.
This was because creating Delta Airlines as a specific manual term would be hard due to the homonyms for Delta. Despite attempts to exclude terms like 'Covid,' 'variant,' and 'dental,' the search results still overrepresented queries related to other “delta” searches that were unrelated to the airline. For example, I could still see some incremental increase in search activity for the Delta variant in July 2021 despite my efforts to remove it and there’s still many more homonyms and related terms for the word “delta”.
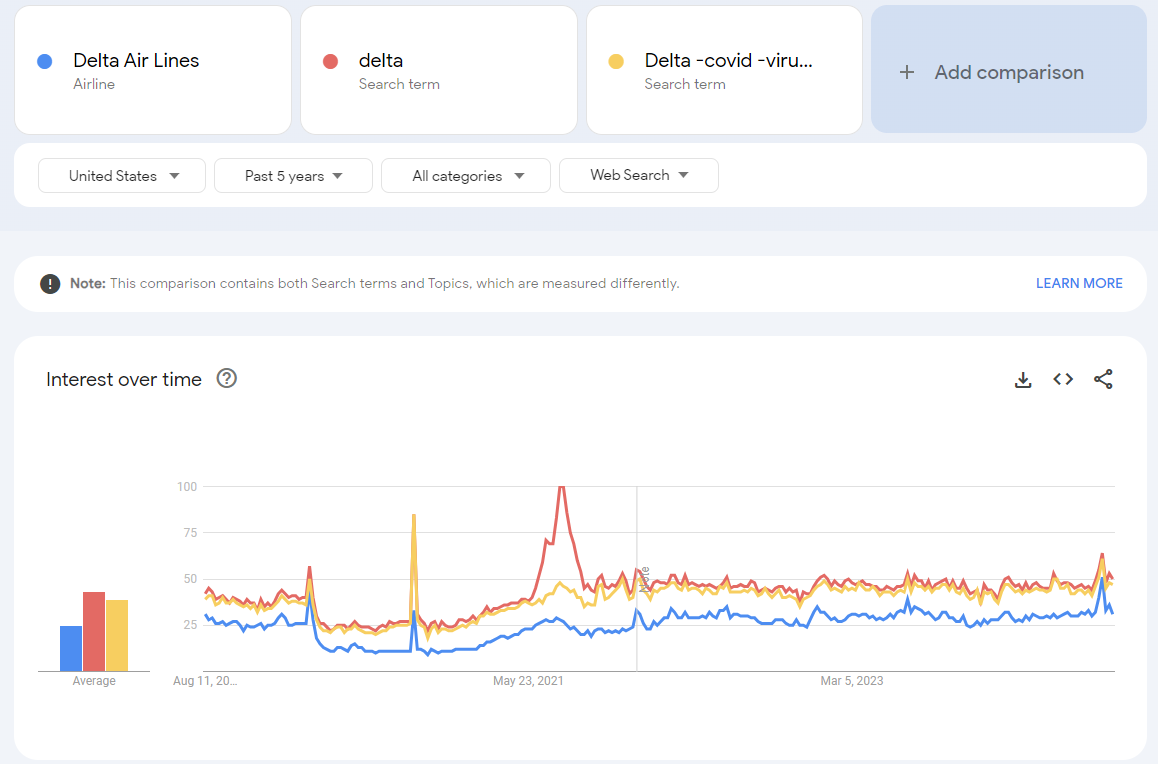
Then I thought about Southwest and how to remove non-airline references to the Southwest region, recipes, etc. Then I thought about American and United and just said, "Nope.”
But the problem with the prebuilt search terms is that they are a black box and not in your control. Sometimes the returned results are just not intuitive or are volatile. They could change their logic and the data will permanently change for better or worse.
The type of topic can also lead to incorrect results. For example, searching “American Airlines” offers three predefined options, two labeled as “topics,” each yielding different results. The “correct one” seems like selecting “American Airlines” tagged as “Airline.”
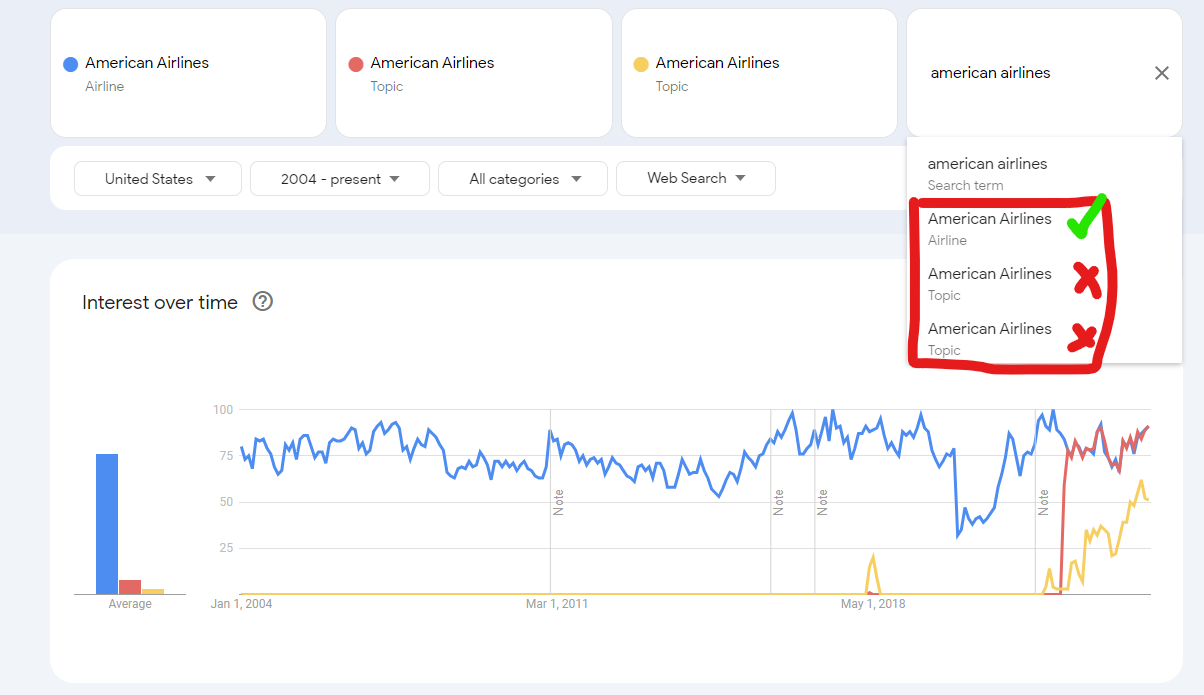
While pre-built terms are available, they should be used cautiously. I’d suggest only using them as a last resort when crafting your own search string is not possible. Using the prebuilt term will not allow for the sample of data to be worked around, which will be covered in Part II.
How should we get search data on “pop music?”
As for creating the term “pop music,” I think we need to throw in the towel and use the pre-built term. Searching for just “pop music” like Rick Beato did led to not many searches being returned because pop music is a specific subset of all pop or music searches (yellow line). Searching for just the word “pop” gives too many searches because of unrelated pop searches (green line). My best effort to remove as many unrelated terms as possible helps, but I’m not confident in the returned values (red line) because the values are still much higher than the pre-built search term for pop music as a music genre as well as the pre-built search for country music, which should be more popular than pop music in the US. I get an error if I try to remove any more unrelated terms from "pop.” So I’m out of options and need to use the prebuilt term.
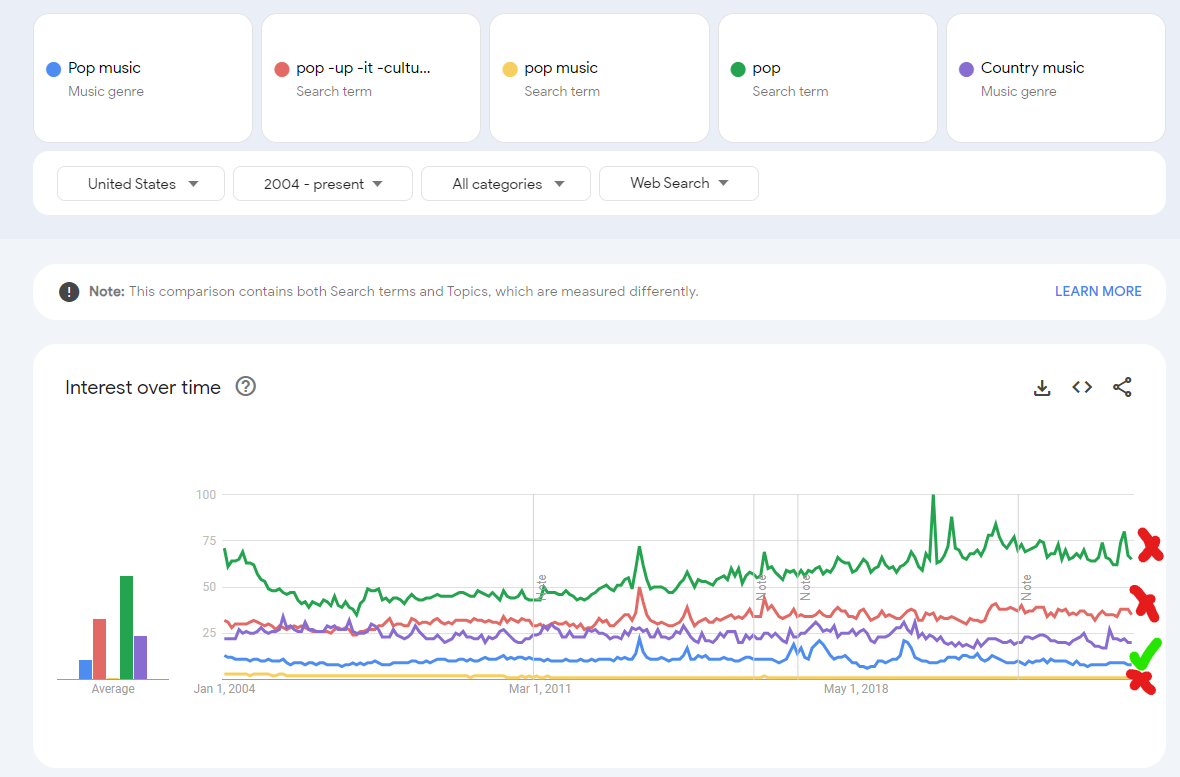
Staying within the page view limits
Before beginning a web mining project, it’s important to be clear about compliance and regulatory best practices. While Google Trends is made available for public use, it is important to say within the limits of page views. Even manually collecting the data with many page views in a short period of time can lead to access being throttled by Google. It’s important to be a good corporate citizen while collecting data from Google Trends by limiting the number of times you query the site in a short period of time.
Stay tuned for Part II:
In the Dataset Deep Dive Series, the format covers the following topics:
Common questions addressed with the data (Covered in Part I)
Underlying Data (Covered in Part I)
Cleaning the Data (Part II)
Enriching the data (Part III)
Limitations to consider (Part III)
Action items to begin using the data (Part III)
I went deep into the underlying data in this part of the deep dive. There’s much more to consider beyond the underlying data related to cleansing and enriching the search data. We’ll cover how to properly clean the data in Part II of the Deep Dive on Google Trends.
And, here are some past Data Deep Dives if you’re interested in getting down to the details of converting raw alternative data into actionable insights:
- Jason DeRise, CFA
Alternative data: Alternative data refers to data that is not traditional or conventional in the context of the finance and investing industries. Traditional data often includes factors like share prices, a company's earnings, valuation ratios, and other widely available financial data. Alternative data can include anything from transaction data, social media data, web traffic data, web mined data, satellite images, and more. This data is typically unstructured and requires more advanced data engineering and science skills to generate insights.
Clickstream data: Clickstream, or web traffic data, refers to the record of the web pages a user visits and the actions they take while navigating a website. Clickstream data can provide insights into user behavior, preferences, and interactions on a website or app.
Very interesting.
When people talk about Google Trends data, I always think of the famous Google Flu Trends research project. Google tried to use their own search data to predict flu outbreaks. The data worked until it stopped working. This was many years ago and I am sure that the data is much more robust now.
But still, there is a potential issue that if people search for something - it can be good or bad. If people search for a specific airline - it could be because there is increase in demand or it could be because there is increase in cancellations. If people seach for "Netflix" - it doesn't always mean that they want to start a new subscription. So the context is important.
I am sure you will talk about it in Part 2.