It Only Takes One Data Point to Disprove an Investment Thesis
Correctly solving the answers to critical investment debates requires a thesis and checkpoints along the way. It also requires testing to prove the opposite view.
Welcome to the Data Score newsletter, composed by DataChorus LLC. The newsletter is your go-to source for insights into the world of data-driven decision-making. Whether you're an insight seeker, a unique data company, a software-as-a-service provider, or an investor, this newsletter is for you. I'm Jason DeRise, a seasoned expert in the field of data-driven insights. As one of the first 10 members of UBS Evidence Lab, I was at the forefront of pioneering new ways to generate actionable insights from alternative data. Before that, I successfully built a sell-side equity research franchise based on proprietary data and non-consensus insights. After moving on from UBS Evidence Lab, I’ve remained active in the intersection of data, technology, and financial insights. Through my extensive experience as a purchaser and creator of data, I have gained a unique perspective, which I am sharing through the newsletter.
This entry in the Data Score Newsletter is going to go deeper into the use of data for investment decision-making, focusing on how to set up an investment thesis1 and incorporate data.
Know the right questions to answer
Design the investment thesis
Design the counter-factual test
Build a data mosaic
Look for new questions to answer
It only takes one data point to disprove an investment thesis. By testing for the counterfactual, it allows for the uncovering of data points that disprove the thesis. That’s a good thing. Knowing that a thesis isn’t playing out as expected earlier than the rest of the market is a win.
The question-driven approach to investing
In the question-driven approach to investing, the focus is on formulating the right questions to understand the investment landscape deeply. This method prioritizes curiosity and critical thinking, encouraging investors to explore beyond surface-level observations. Techniques like the “5 Whys,” “Question Bursts,” and “Jobs to Be Done” interviews are employed to extract nuanced insights.
This approach is crucial in the financial markets, where understanding the core issues and underlying trends, which are constantly changing, influences investment decisions.
For more details, check out this prior entry in the Data Score Newsletter:
Once you have the right questions to answer, formulating a thesis is the next step.
Design your thesis
“Everyone is right in the long term” is a nice way of saying “the investment lost money because the timing was estimated incorrectly.”
What is your opinion on what the answer is? Write it down so you don’t let confirmation bias2 and hindsight bias3 shift your recollection of the original thesis to match the reality that happens later.
Here are some key factors to consider when setting up your investment thesis:
Measurable Outcomes: Define specific, quantifiable goals that your investment seeks to achieve. This clarity enables you to track progress effectively and make data-driven decisions. It’s important that the measures directly relate to the questions you are answering. Avoid accidentally substituting easier questions to answer. It is ok to, and encouraged to, break the big question into smaller, answerable questions, so long as the questions are directly related to the big question. Make sure to be fully exhaustive with the smaller questions, noting questions that can’t be answered with measurable outcomes yet.
Time Frame for Outcome: Establish a realistic timeline for your investment goals. This aids in setting appropriate expectations and aligning strategies with market cycles. “Everyone is right in the long term” is a nice way of saying “the investment lost money because the timing was estimated incorrectly.”
Dependencies and Drivers: Identify key factors that could impact your investment thesis. Understanding these elements helps in anticipating market shifts and adjusting strategies accordingly. What are the underlying factors that explain movements in the measurable outcomes? What are the constraints that limit the ability to achieve the outcome? What are the reasons why the measurable outcome hasn’t been achieved already?
Checkpoints on the Correct Path: Implement regular reviews to ensure that your investment is on track. These checkpoints allow for adjustments based on new data or changes in market conditions.
What’s in the valuation already? To generate alpha4, your thesis must turn out to be true and be recognized in the share price in the future, but importantly, the current share price does not yet reflect your thesis.
For example, does your investment thesis predict the long-term adoption of AI by businesses to generate new disruptive products and improve productivity? Guess, what? So does everyone else’s thesis. That’s probably already included in the share price. Sure, you could jump on the bandwagon and have beta5 exposure to the theme. But if you want to outperform the market, you’ll need to have a different view than consensus6.
Differences vs. consensus views could be entirely different or nuanced. It’s important to be able to explain the differences versus consensus, and the differences need to be aligned with your investment question and thesis. If your difference of view vs. consensus is answering a different investment question than the critical investment debate, it won’t matter if your answer is correct, as it’s answering the wrong question.
Let’s try the above out with a generic example
If we were to take that generic thesis from above about the “long-term adoption of AI by businesses to generate new disruptive products and improve productivity” and build it out properly, the thesis could include:
Measurable Outcomes: Focus on specific and trackable metrics, such as the aggregate revenue of AI businesses, to gauge market reach, or monitor the reduction in SG&A7 as a percentage of sales in industries adopting AI to see the productivity gains. Setting concrete, quantifiable targets for these metrics enhances the precision and accountability of your investment strategy.
Timeframe of outcome: For the measures you estimate, include the time frame that it will be achieved. For example, Bloomberg Intelligence published on June 1st, 2023 “With the influx of consumer generative AI8 programs like Google’s Bard and OpenAI’s ChatGPT, the generative AI market is poised to explode, growing to $1.3 trillion over the next 10 years from a market size of just $40 billion in 2022, according to a new report by Bloomberg Intelligence (BI).” https://www.bloomberg.com/company/press/generative-ai-to-become-a-1-3-trillion-market-by-2032-research-finds/
Dependencies and drivers: What is also needed to achieve this outcome? For one, the total addressable market9 estimate for AI shouldn’t be higher than the total tech spend (and it probably shouldn’t be anywhere close to total tech spend, unless you really believe 100% of tech spend will be AI spend in the future). The participants in the industry can’t generate aggregate revenue higher than the total addressable market (you’d be surprised how many professional investors forget that market share is a zero-sum game). These are some examples of modeling limitations. Then there are real-world dependencies and drivers such as the available computing power to support future AI applications, in the form of advanced processors (GPUs10), or a prediction of new AI capabilities that use less computing power per task. There are also funding questions, as AI technology is not likely to be available for free. How much can be spent on AI without affecting company solvency? There are also potential regulatory constraints imposed by governments that limit growth. And, don’t forget, the AI applications have to actually be useful, which requires relevant, accurate data to power the AI to drive new valuable products and improve productivity.
Checkpoints: If you share Bloomberg Intelligence’s view, the estimated outcome is not happening overnight, and there is a growth path to achieve the target. The article talks about which industries would drive the growth and their trajectory. These would act as future checkpoints to see if the thesis is playing out. This is where its important to be honest with ourselves as the checkpoints arrive. If the path isn’t playing out as expected, the temptation will be to backload the estimate, expecting higher growth in the future to compensate for slower growth in the near term.
What’s in the valuation now? You need to be able to state how your view is different from the consensus view.
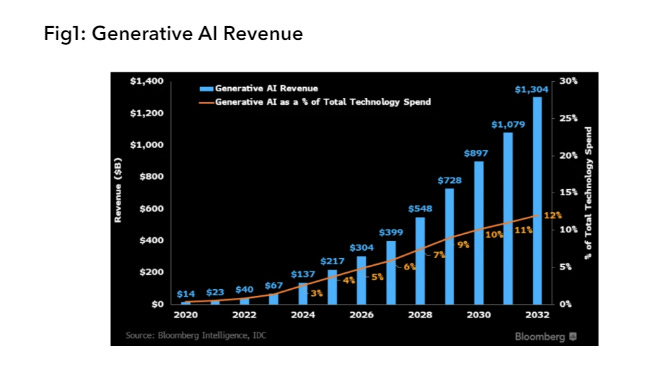
Testing for the counterfactual
It only takes one data point to disprove an investment thesis. By testing for the counterfactual, it allows for the uncovering of data points that disprove the thesis. That’s a good thing. Knowing that a thesis isn’t playing out as expected earlier than the rest of the market is a win.
Continuing with the line of thinking in setting the thesis above,. Imagine scenarios where the dependencies and drivers do not play out. What would that scenario look like? What would it look like if AI applications were not generating value for users? Or what would it look like if processing power was a constraint? These would set up a downside scenario that could be tracked with checkpoints. Having this set up at the beginning of the process gives a more tangible way to judge which scenario is more likely to play out.
It shouldn’t just be dark-sky scenarios. What do blue-sky scenarios look like? How would we know the checkpoints were pointing to faster adoption or a larger TAM than the base case?
The scientific method's focus on testing the null hypothesis11 offers a valuable parallel for investment strategies. While applying statistical P-value12 tests directly may not be practical, adopting the underlying mindset of rigorously challenging assumptions can significantly enhance the robustness of investment analysis (unless the goal is interpreted as ensuring the p-value test passes by p-value hacking the analytics to make sure the null hypothesis is rejected, which would completely miss the point of testing for the counter-factual).
Build the Data mosaic
Constructing a data mosaic is about piecing together various data points and sources to form a complete picture of the investment environment. This process involves collecting, analyzing, and synthesizing information from diverse sources to gain comprehensive insights. The aim is to create a robust framework that supports your investment decisions, taking into account different market dynamics and scenarios.
Not only would you want to collect data on specific measurable outcomes, but you would also want to measure the dependencies and drivers.
In addition to the total addressable market or the SG&A ratio of companies adopting AI, measure drivers such as the number of businesses with AI deployed in their tech stack, the number of job listings seeking AI-related skills, the availability of GPU inventory for small businesses to purchase, the sentiment on AI applications in the press, search activity for AI, app usage13 for apps featuring generative AI, software reviews, clickstream data14 for AI web applications, patents data, etc.
Check out this older Data Score Newsletter for some additional ideas on data to track AI adoption. https://thedatascore.substack.com/p/nvidia-how-could-alternative-data
And in the graphic below, I share some ways to map common alternative datasets to different KPIs and use cases. These common alternative data types could be used to think about testing different types of investment thesis:
Look for new questions to answer
It’s important to consider that the market is forward-looking by nature. Once a debate is answered, the market values that outcome in the share price. Therefore, the volatility of the price will be driven by unanswered questions. It’s important to constantly test if the question that is being answered by the data is the same question the market is asking. If not, answering the wrong question accurately is likely to lead to disappointing outcomes in investing.
Testing for the counterfactual involves exploring scenarios where your investment thesis could be proven wrong. This process is vital for identifying potential risks and weaknesses in your strategy. It involves considering alternative viewpoints and challenging assumptions to ensure a comprehensive understanding of the investment landscape.
A brief epilogue on my sell-side analyst career
The advice about the investment thesis process is a culmination of what I learned about investing, beginning back in the Syracuse University Investment Club, working at Sanford Bernstein as an associate, becoming a senior analyst at UBS, and then working with the best analysts at UBS from my early seat in UBS Evidence Lab.
As a sellside15 analyst, I was always quite proud of my earnings estimates rating on Starmine compared to competing sellside analysts covering the same company. I was typically highly rated on estimates. But it depended on the year for my stock-calling performance. But if you look at my last year as a covering analyst, it was about as bad as it could be. I plan on sharing more details from that year in the newsletter. The high-level summary is that I had all the estimates right and the answers to many questions right, but the stock calls were wrong.
With the benefit of seeing the role of the analyst through my seat in Evidence Lab, I was able to see the missing part of my process.
The questions that are to be answered have to be the right ones to answer—the ones the market actively wants answered. When I had great stock-calling years, the questions I was answering were the right ones to answer. But when the market’s questions change, it’s important to pause and reset the thesis and weigh in the various data points accordingly.
Being clear about what’s in the current valuation and how my views and estimates differed from consensus in relation to the valuation.
The process of leveraging checkpoints to make sure the thesis path is still there or if the reality of the data is revealing a different story.
Embrace the early disproving of a thesis as an opportunity to pivot your perspective and strategy. The goal isn’t to be the smartest analyst at the beginning of the thesis process. The goal is to get the investments right.
Going back to my days at Syracuse University, all I wanted to be was a sell-side analyst, and I was thrilled to have achieved the goal so early in my career. In my journey as a data professional, I am now even more passionate about empowering others' investment success through my insights into data-driven investing and the development of scalable data products.
- Jason DeRise, CFA
Investment Thesis: A clear, definable idea or set of ideas that outlines an investor's expectations and reasons for the potential outcome of an investment.
Confirmation Bias: A tendency to search for, interpret, favor, and recall information in a way that confirms one's preexisting beliefs or hypotheses.
Hindsight bias: Hindsight bias is a psychological phenomenon that allows people to convince themselves after an event that they accurately predicted it before it happened. This can lead people to conclude that they can accurately predict other events. Hindsight bias is studied in behavioral economics because it is a common failing of individual investors. https://www.investopedia.com/terms/h/hindsight-bias.asp#:~:text=Hindsight bias is a psychological,can accurately predict other events.
Alpha: A term used in finance to describe an investment strategy's ability to beat the market or generate excess returns. A simple way to think about alpha is that it’s a measure of the outperformance of a portfolio compared to a pre-defined benchmark for performance. Investopedia has a lot more detail https://www.investopedia.com/terms/a/alpha.asp
Beta: In finance, beta is a measure of investment portfolio risk. It represents the sensitivity of a portfolio's returns to changes in the market's returns. A beta of 1 means the investment's price will move with the market, while a beta less than 1 means the investment will be less volatile than the market. In the context of data, beta refers to the data’s ability to explain the market’s movements because the data is widely available and therefore fully digested into the share price almost immediately. This level of market pricing efficiency means there’s not much alpha to be generated, but the data is still needed to understand why the market is moving.
Consensus: “The consensus” is the average view of the sell-side for a specific financial measure. Typically, it refers to revenue or earnings per share (EPS), but it can be any financial measure. It is used as a benchmark for what is currently factored into the share price and for assessing if new results or news are better or worse than expected. However, it is important to know that sometimes there’s an unstated buyside consensus that would be the better benchmark for expectations.
SG&A (Selling, General, and Administrative Expenses): The category of selling, general, and administrative expenses (SG&A) in a company's income statement includes all general and administrative expenses (G&A) as well as the direct and indirect selling expenses of the business. This line item includes nearly all business costs not directly attributable to making a product or performing a service. SG&A includes the costs of managing the company and the expenses of delivering its products or services. https://www.investopedia.com/terms/s/sga.asp.
SG&A Ratio: A financial metric used to measure the selling, general, and administrative expenses as a percentage of total sales.
Generative AI: AI models that can generate data like text, images, etc. For example, a generative AI model can write an article, paint a picture, or even compose music.
Total Addressable Market (TAM): Total addressable market (TAM), also called total available market, is a term that is typically used to refer to the revenue opportunity available for a product or service. TAM helps prioritize business opportunities by serving as a quick metric of a given opportunity's underlying potential. https://en.wikipedia.org/wiki/Total_addressable_market
GPUs: An acronym for "Graphics Processing Units." These are specialized electronic circuits designed to rapidly manipulate and alter memory to accelerate the creation of images in a frame buffer intended for output to a display device.
Null Hypothesis: In scientific research, the null hypothesis is the claim that the effect being studied does not exist. Note that the term "effect" here is not meant to imply a causative relationship.The null hypothesis can also be described as the hypothesis in which no relationship exists between two sets of data or variables being analyzed. If the null hypothesis is true, any experimentally observed effect is due to chance alone, hence the term "null." In contrast with the null hypothesis, an alternative hypothesis is developed, which claims that a relationship does exist between two variables. If the sample data is consistent with the null hypothesis, then you do not reject the null hypothesis. https://en.wikipedia.org/wiki/Null_hypothesis
I would add that this does not mean the null hypothesis is true, but it does hurt the case that the hypothesis is true. Likewise, failing to reject the null hypothesis should only increase confidence that the relationship between the data points and the hypothesis is true. As the Wikipedia definition states, this does not mean there is proof of a causal relationship. But, so long as we fail to reject the null hypothesis, our hypothesis could still prove to be true. Keep on setting up new hypothesis tests, as this is not the end of the process in practical terms, especially in investing.
P-value: In null-hypothesis significance testing, the p-value is the probability of obtaining test results at least as extreme as the result actually observed, under the assumption that the null hypothesis is correct. A very small p-value means that such an extreme observed outcome would be very unlikely under the null hypothesis. Even though reporting p-values of statistical tests is common practice in academic publications of many quantitative fields, misinterpretation and misuse of p-values is widespread and has been a major topic in mathematics and metascience. In 2016, the American Statistical Association (ASA) made a formal statement that "p-values do not measure the probability that the studied hypothesis is true, or the probability that the data were produced by random chance alone" and that "a p-value, or statistical significance, does not measure the size of an effect or the importance of a result" or "evidence regarding a model or hypothesis."That said, a 2019 task force by ASA has issued a statement on statistical significance and replicability, concluding with: "p-values and significance tests, when properly applied and interpreted, increase the rigor of the conclusions drawn from data." https://en.wikipedia.org/wiki/P-value
App analytics: This refers to the measurement of user engagement and usage patterns within a mobile app. It can help identify how users interact with the app, what features are most used, and where users are facing issues.
Clickstream data: Clickstream, or web traffic data, refers to the record of the web pages a user visits and the actions they take while navigating a website. Clickstream data can provide insights into user behavior, preferences, and interactions on a website or app.
Buyside typically refers to institutional investors (Hedge funds, mutual funds, etc.) who invest large amounts of capital, and Sellside typically refers to investment banking and research firms that provide execution and advisory services (research reports, investment recommendations, and financial analyses) to institutional investors.