From Data to Decisions: The Power of Diverse Data and Analytical Tools
Diversify your analytical toolkit to uncover deeper insights and drive better decision-making.
Welcome to the Data Score newsletter, composed by DataChorus LLC. The newsletter is your go-to source for insights into the world of data-driven decision-making. Whether you're an insight seeker, a unique data company, a software-as-a-service provider, or an investor, this newsletter is for you. I'm Jason DeRise, a seasoned expert in the field of data-driven insights. As one of the first 10 members of UBS Evidence Lab, I was at the forefront of pioneering new ways to generate actionable insights from alternative data. Before that, I successfully built a sell-side equity research franchise based on proprietary data and non-consensus insights. After moving on from UBS Evidence Lab, I’ve remained active in the intersection of data, technology, and financial insights. Through my extensive experience as a purchaser and creator of data, I have gained a unique perspective, which I am sharing through the newsletter.
To drive better decision-making, it's crucial to diversify your analytical toolkit and start with the right questions. This approach helps uncover deeper insights and avoids the pitfalls of overrelying on familiar tools.
I was recently invited as a guest to one of my favorite podcasts, the Analytics Power Hour. The most enjoyable podcasts to listen to are those that feel like you get to listen in on a conversation between people who enjoy spending time together talking about something they deeply care about. The hosts, Val, Julie, and Tim, made it easy to have fun while nerding out about data and analytics! Thanks for having me on!
In this entry of The Data Score, I dig a bit deeper into the theme of building a mosaic of insights from a wider range of data that can improve the decision-making process.
Here are 4 key points from the conversation:
Pitfalls of relying on limited tools.
Importance of diverse analytical tools.
The importance of asking the right questions.
Role of design thinking and team diversity.
I then share some examples and practical tips before sharing the “Last Calls” from the podcast.
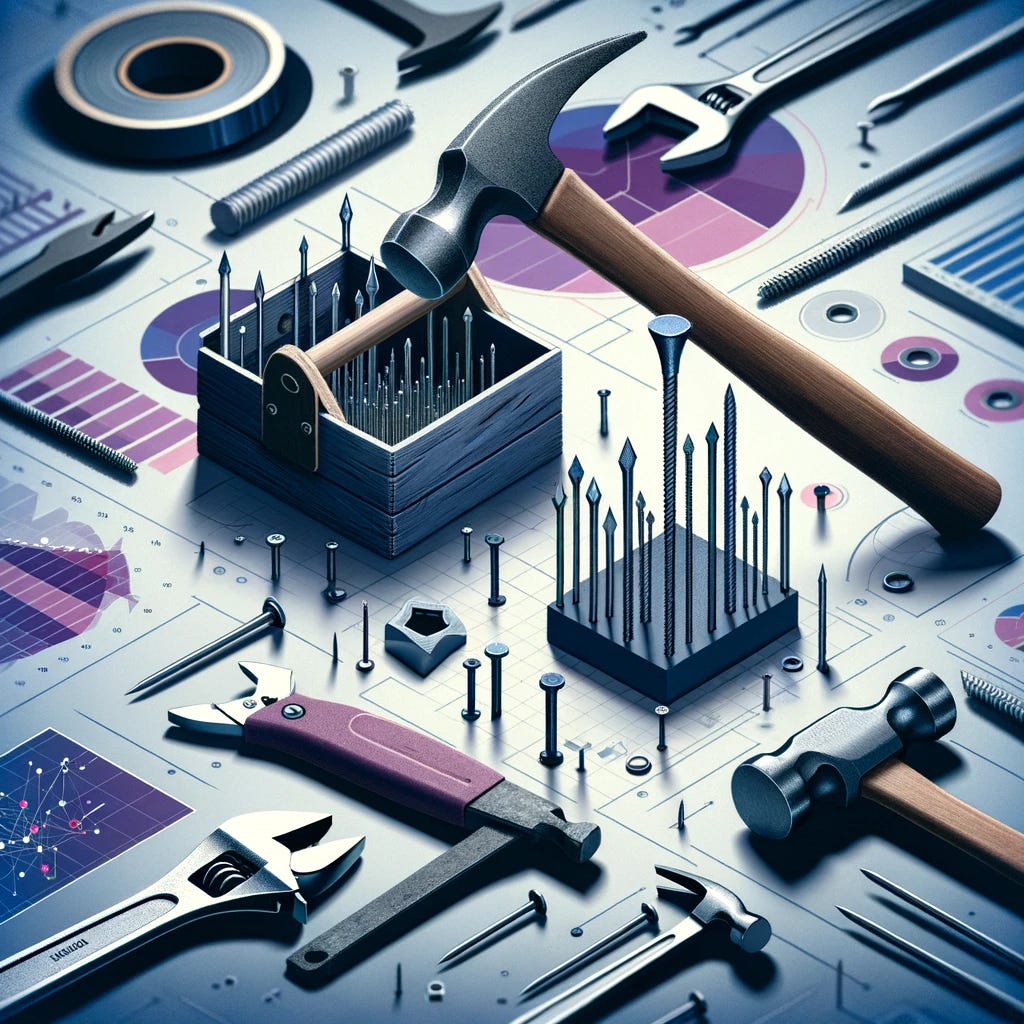
The pitfalls of relying on just a hammer and nail
Analytic hammers and nails are when an analyst or decision maker sticks with one or two data points and analytic frameworks to make decisions, regardless of the question that needs to be answered.
A carpenter selects the right tools and materials for each task. They wouldn't use a power saw for intricate molding work. I’d like to note that one of my closest friends, who is a former carpenter and is currently one of the most prominent experts in alternative data1, is now most likely actively typing a text to me explaining how bad my analogy is.
The same applies to data. Specific data and analytic processes may be perfect for one outcome but not for another. Yet, people often revert to familiar tools regardless of the question.
This bad habit has two main risks:
It causes tunnel vision and limits insight.
Overfitting2 data beyond its appropriate use can lead to wrong conclusions.
Hammers are great, but not for every task. Surveys are great, but not for every insight.
An example is that some people love surveys. Surveys are great for understanding intentions and perceptions of the why behind behaviors that can be measured with other data sources. A well-crafted survey that considers the psychology behind the process of answering survey questions and how humans recall past events or think about future intentions can unlock breakthrough insights. But surveys are less useful for measuring actual behaviors, especially when there is a major change in the world that could lead to new behaviors. Think back to November 2022, when ChatGPT began to gain its initial popularity with the general public. The big question at the time might have been, “Will large language models displace Google search?”
Someone who treats surveys as the solution to every problem might be inclined to ask consumers or business professionals questions to understand if they will switch from Google to ChatGPT. The quantitative intent of the survey population is interesting, but it is not the same as measuring the behavior of consumers and businesses.
The use of the full tool kit would include monitoring search activity, clickstream data3, and app usage data (app analytics4). These measure the behaviors and would reveal more specific answers to know if switching is happening. Additional datasets can confirm if survey intentions translate into real behaviors. Early surveys on ChatGPT 3 would show different, incomplete results compared to actual usage, risking missed insights and incorrect conclusions.
Repeatable surveys over time capturing changes in intention is useful in the right circumstances because changes in real intentions is the first step before actual behavior changes. So, I don’t want the takeaway from anyone to be that “Jason doesn’t like surveys!” Surveys are great for the right use cases.
Hammers and nails in the corporate world
Having always been in financial markets, I was curious about the corporate world's approach. I expected similar competitive pressure to spur the use of non-traditional data for new insights.
Tim Wilson shared his corporate experience on the podcast:
"I feel like the corporate side just gets really stuck in, let’s just go deeper and deeper and deeper in the data that we have and doesn’t look out. They don’t have that kind of competitive pressure. They may think they do, but they don’t. And therefore, there’s not an incentive."
"I have this one simple example, which was a former coworker of all three of ours. Sam Burge was working on it with the home builder, and it was just one where she was trying to figure out if stuff was having an effect, stuff that was being done. And she was like, well, what if we looked at housing starts data?"
"And it was like, well, that’s amazing. And she was like, 'Can I go get it? Can I get it relatively quickly? Does it actually seem to help?' As I recall, there was a little bit of hesitation from some parties, saying, why are you bringing in this other data set?"
These quotes reflect Tim's insights into how corporate environments often struggle with integrating alternative data, using real estate data as an example of how corporations might overlook useful external data sources due to a lack of urgency or competitive pressure.
These insights show how corporate environments struggle with integrating alternative data. Tim used real estate data as an example of overlooked external sources due to a lack of urgency or pressure.
The Importance of Diverse Analytical Tools
It is not easy to transition to this approach of using a full toolkit for problem solving, leveraging a design thinking approach to problem solving, and properly focusing on the important questions to answer. Changing the culture takes time. I shared some thoughts on what I’ve seen in the past in my career:
*I think in the beginning, it was a bit harder to get people to think this way.
When it was working well, we would try to get our stakeholders to open up about what they were actually trying to solve. You know, get them to talk more about outcomes.
Trying to tease out of them what they actually were after. And to me, it was more about what they didn’t know. More so than what they thought the answer was. I wanted to know both. And once you could find what they didn’t know or what the uncertainty was, we had a way of answering it that was different, and then we had their attention, as long as they were open-minded about it.
And so over time, we found early adopters showed value, would create a bit of social proofing, or FOMO, and others would say, “Oh, wait, that question is similar to my question. Can you help on this one?”*
Social Proofing5 FOMO6
Be specific on the outcome needed
As discussed above, the time spent understanding the right question to be answered, as well as what is known and unknown about the potential answers to the question, will help make the data sourcing and analytics laser focused on achieving results that matter. Building a data product well means that the data product provides a trusted insight into an economic outcome such that the user of the data product simply needs to make a decision and not do more analytics to get to the answer.
Be data source and analytic tool agnostic
With clear outcomes in mind, deciding on the best solution in our design thinking approach means we need to be data source and analytics tool agnostic. We shouldn’t care about which data, platforms, or techniques we are most comfortable with or “how we always do it.” This is where first principle thinking is most important. “How should things be?” Not “How has it been?”
Once again, the benefits of a diverse team come into play, as each person’s unique skills can be leveraged to get the outcome needed. There are very few unicorns who are experts in everything.
Start small and hand crafted, learn, and adapt
When there's an idea to solve a problem with data, it's crucial to try it end-to-end. Work quickly on an end-to-end data product, making the initial product a substantial vertical slice of the full analysis. In some cases, the vertical slice of the full analysis is very thin, but this is still needed to be able to try, get feedback, and adapt.
It’s a universal experience in data and analytics to have spent time on a solution only to find out the final metrics planned at the beginning are not exactly useful yet. It wasn’t intended to be “just interesting,” but once it was delivered, it became obvious that it wasn't useful yet. So we just need to go back to the drawing board and try again, quickly.
Take the vertical slice that received feedback and rework it. Show it to another stakeholder to gauge its usefulness. Take their feedback, adapt it, and show to another stakeholder. Revisit the original stakeholders with the improvements and see if they are starting to say, “Can I use this now? When can this be done for more verticals?” Once they can’t wait to have it in their hands, you should feel more confident that there’s product/market fit7.
Do a small test and see if it works. If it worked, try, try again, try again. And eventually, if we found product market fit, we really tried to scale it up.
The Power of Asking the Right Questions
Readers of the Data Score know that good analytics starts with asking the right questions first.
The article explained the importance of asking the right questions to drive effective, data-driven decision-making. Key points include:
Importance of Questions: Effective decision-making begins with asking the right questions to fully understand problems before jumping to solutions. Techniques like the "5 Whys," "Question Bursts8," and "Jobs to Be Done9" interviews are highlighted.
Clairvoyance Test: Ensure questions are well-phrased, measurable, and relevant. Questions should provide information that would lead to actual decisions being made. The answers, no matter what they end up being, would be usable.
Breaking Down Questions: Large, complex questions should be broken down into smaller, answerable questions. This helps build a comprehensive understanding.
Practical Methods:
5 Whys: Iteratively asking "why" to dig deeper into the root cause of a problem. But consider this pro-tip: don’t actually use the word "why.”
Question Bursts: Generating numerous questions in a short time to uncover deeper understanding.
Jobs to Be Done: Understand user goals and outcomes to design better products and services.
Outcome-Based Research: Focus on user outcomes rather than specific product features to create solutions aligned with user needs.
Narrative Importance: Understanding and explaining the current situation ("What's going on here?") before predicting future outcomes. Discuss potential data outputs to tell a story that explains the action to be taken in various scenarios of how the questions could be answered.
By starting with questions, teams can ensure their work aligns with desired outcomes, enabling more effective use of data for decision-making.
How do we know the right questions to prioritize answering?
It’s important to focus on specific business problems. The business strategy should drive the data strategy. For investors, investment questions drive the data strategy.
But we can’t answer all questions, we need to focus on the critical, high-impact questions. Avoid working on merely interesting questions. "Interesting" isn’t good enough. We discussed this on the podcast, and I shared this perspective.
We learned along the way that if we heard "interesting," that was “a hard no, we’re not doing it.” And we would tell you, “It’s interesting, but it’s not good enough.” It’s got to be “have to have,” otherwise we’re going to deliver something to you and you’re going to be like, “Okay, what do I do with that?”
How do we know if it’s actually important? Here’s another podcast quote:
This is just one of the learnings along the way about how we make sure that we’re generating value with the work that we’re doing. And what we found to be the most important piece of it was not starting with the data, it was starting with the questions. And in particular, it’s questions where you imagine you have the answer; would that answer actually change your opinion?
We didn’t get the analyst to use the full scientific method. Val, I think your A/B testing was way more scientific than anything that the research analysts would do. But we would try to get them into that mindset where, “Okay, I think x, y, and z are going to happen for this company, and if this data came through and disproved it, that would actually be a huge value because now we know that the data is saying to go in a different direction.” And if it fails to disprove it, “Great, I can keep my view.” All it takes is one data point to really break an investment thesis.
And the best analysts that I worked with were really good about just following the data and not falling into any biases about what their views are.
Investment Thesis10
Role of Design Thinking and a Team Diversity
A key aspect of ensuring creativity and rigor in decision-making with a full data toolkit is how ideas are generated. This involves a “design-thinking” approach to brainstorming and having many diverse perspectives from various backgrounds, disciplines, and experiences in the room contribute ideas.
What is design thinking?
Design thinking is a user-focused, creative problem-solving process. It involves deeply understanding the customer experience to generate many ideas before converging on solutions.
IDEO, known for partnering with Apple and other major companies, is famous for applying and perfecting this approach.
Here’s what Tim Brown, Executive Chairman of IDEO, has to say about design thinking:
Design thinking is a human-centered approach to innovation that draws from the designer’s toolkit to integrate the needs of people, the possibilities of technology, and the requirements for business success. — TIM BROWN, EXECUTIVE CHAIR OF IDEO https://designthinking.ideo.com/
IDO talks about customer-focused design that emphasizes desirability, viability, and feasibility.
The process starts by creating choices. Once enough choices are generated, the focus shifts to converging on the best ideas.
Diversity within the team expands the options and leads to better choices
The human centric nature of design thinking, requires empathy for the end users of any product (data or otherwise). There’s no such thing as an average user of a product. In reality, end user of a product is diverse. Having a diverse team increases empathy for the user.
Diverse perspectives bring more ideas to the table during the creating choices phase of design thinking. The more different ideas there are, the better. Take a “yes, and” approach to brainstorming by building on ideas with more ideas. Brainstorming sessions with a diverse group lead to so many surprising and inspiring ideas that it’s easily the most exciting part of the job, in my opinion. Everything is possible. No idea is to crazy. People with different experiences and perspectives coming together create extraordinary possibilities.
With a wide range of ideas and end-user focus, we can narrow the list to what's viable, feasible, and still desirable.
On the Analytics Power Hour podcast, I talked about the experience I had at UBS Evidence Lab, where I saw firsthand the power of this approach.
We had all sorts of different backgrounds. There were people with psychology backgrounds. There were climatologists, geospatial experts, and experts at social media data. And so we brought this unique collection together and it was very much a design-thinking model. We just wanted to get as many ideas on the table as possible and then filter down to what we actually thought could be done.
Real-World Examples
On the podcast, we discussed examples of alternative data requiring the creation of applications of technology and analytics to turn the raw data into insights.
Vessel Tracking for Trade Analysis
Tim Wilson brought up the idea of vessel tracking as a type of alternative data. Here’s what I had to say about it.
It’s a great example because it’s exhaust data for another purpose. So, all the vessels have tracking devices on them, and it’s reading out the speed, it’s reading out their geocoordinates, it’s telling how deep the vessel’s sitting in the water, and this is really to make sure the vessel doesn’t go missing.
If you take that data set and you turn it on its side and you aggregate it for specific questions around what’s happening with global trade, what’s happening with maybe specific commodities because you can combine that data with data about the different ports and you can know certain ports are oil ports or iron ore. So, we actually did that. That was one of the many things that we tried to build and successfully build was one of the more popular products that we created using these alternative data sets.
I wrote a deep dive article in June 2023 about how to turn the exhaust data11 of vessel locations into insight ready data.
Web Scraping for Consumer Insights
On the podcast, I discussed how freely available information across the web could be extracted, cleansed and enhanced to answer investment questions.
We discussed some consumer sector applications:
We were using web robots to go across Amazon and other retailer sites every week, collecting as much or if all of the assortments that were available, the prices and a whole bunch of attributes about the products, the brand name, the product, and the category. By collecting every week, going through the full assortment, seeing the prices, and understanding how we were basically able to reverse engineer prices and get back into what was happening with inventory.
We also discussed using web-mined data for real estate applications.
We were scraping the prices from leading property portals in multiple countries and analyzing it the way a residential real estate analyst would and seeing that shift in inventory levels.
I also did a deep dive write up on web mined data use in the apparel sector in July 2023:
I’m glad in the conversation we did talk about web robots in the context of being compliant with regulations and also being a good corporate citizen.
Geospatial Data
We also discussed the use of various geospatial data12, including monitoring aggregated, anonymized cell phone locations. There was also discussion of freedom of information act (FOIA)13 requests to get address change data (anonymized and aggregated).
We discussed monitoring behaviors during the early pandemic period through recovery to the then-unknown "new normal." This was a time period when all the traditional data was too slow to help. The algorithms in the models could not work because the historic data used to train the models did not include any situations like this.
Many companies made data available on population behaviors, but these data sets lacked historical depth. Even with history, the unique events of the time altered behavioral patterns. A clear understanding of data usage and the right questions enabled extracting value from limited data history.
Combining this data with clickstream, app, transaction, and search data created a mosaic of behaviors, providing quicker visibility than traditional data sets at the time.
Practical Tips
Identify clear data objectives with insight-ready metrics that answer specific questions.
Understand data limitations.
Test and validate data.
Combine multiple data sources.
Conclusions and “Last Calls”
Leveraging a diverse analytical toolkit and focusing on the right questions can significantly enhance decision-making. By avoiding the pitfalls of limited tools and embracing diverse perspectives, organizations can uncover deeper insights and drive better outcomes.
Here are two main takeaways:
Lean into the importance of a diverse toolkit for better insights.
Start with the right questions and combine multiple data sources.
Caveats
There is an important caveat to call out about seeking the use of the full toolkit. We don’t want to use different data and analytical techniques just because they might be cool or fun (ok, cool or fun to us data folks) Podcast co-host Tim Wilson wrote an article about the tyranny of too much data, which I totally agree with. Data has to be outcome-driven, not just new data for the sake of new data. Raw data is a cost, not a benefit in and of itself. The insights have value.
The article warns against the obsession with data collection, highlighting hidden costs in initial setup, maintenance, and analytical complexity. Excessive data can lead to significant expenses and inefficiencies. Organizations should focus on business priorities, align on key problems, and treat ideas as hypotheses to manage data costs effectively.
https://thefocus.factsandfeelings.io/the-tyranny-of-more-data-f82271fb54b6
“Last Calls”
I had a blast discussing data and analytics with Val, Julie, and Tim on the podcast.
I also want to call out podcast co-host Val Kroll’s article from April 2024, “The Data-Driven Toolbox is More Than Just a Hammer.” The article looks at how to decide the right approach in marketing analytics. Val and I worked together at UBS for a few years. She’s an expert on A/B testing14, amongst many other things. Those who stick around for the end of the podcast for “Last Calls” will hear about how Val and I were members of the Evidence Lab “Fun Police,” and we share some advice to not host an “Office Olympics” event.
https://thefocus.factsandfeelings.io/the-data-driven-toolbox-is-more-than-just-a-hammer-90e5633389b3
Here are some other “last calls” from the podcast, which were shared in the show notes of the episode:
Low-Key Data Happy Hour (Westchester) organized by Jason Taylor from Automated Data (ADI)
**Low-Key Data Happy Hours (NYC)** organized by Ethan Aaron from Portable
**Alternative Data Happy Hours in NYC** organized by Jordan Hauer from Amass Insights
The Focus, a Medium publication brought to you by facts & feelings
The Arc Browser and, in particular, the Easel feature
**Data science jobs at Liberty Mutual** (overall)
And bonus, bonus last call
The Analytics Power Hour podcast followed up on the theme by interviewing Simon Jackson from Hypergrowth Data in an episode titled “#246: I've Got 99 Analytical Methodologies and Need to Pick Just One.“ The podcast episode, which recently dropped, discusses how to choose the right analytic tool. I really enjoyed listening to this episode.
- Jason DeRise, CFA
Alternative data: Alternative data refers to data that is not traditional or conventional in the context of the finance and investing industries. Traditional data often includes factors like share prices, a company's earnings, valuation ratios, and other widely available financial data. Alternative data can include anything from transaction data, social media data, web traffic data, web mined data, satellite images, and more. This data is typically unstructured and requires more advanced data engineering and science skills to generate insights.
Overfitting: When a model matches the training data very well when back-tested but fails in real-world use cases when the model is applied to new data.
Clickstream data: Clickstream, or web traffic data, refers to the record of the web pages a user visits and the actions they take while navigating a website. Clickstream data can provide insights into user behavior, preferences, and interactions on a website or app.
App analytics: This refers to the measurement of user engagement and usage patterns within a mobile app. It can help identify how users interact with the app, what features are most used, and where users are facing issues.
Social Proof (or informational social influence): “a psychological and social phenomenon wherein people copy the actions of others in choosing how to behave in a given situation. The term was coined by Robert Cialdini in his 1984 book Influence: Science and Practice. Social proof is used in ambiguous social situations where people are unable to determine the appropriate mode of behavior and is driven by the assumption that the surrounding people possess more knowledge about the current situation.” - Wikipedia definition: https://en.wikipedia.org/wiki/Social_proof In the context of data products, social proofing means demonstrating the value and success of the product by showcasing the positive experiences and endorsements of satisfied users, which in turn can help convince potential clients to adopt the product.
FOMO: Fear Of Missing Out
Product/Market Fit: The ability of a product to meet the needs of customers, generating strong and sustainable demand for the product. This term refers to the point at which a product or service has been optimized to meet the needs and preferences of its target market, resulting in strong customer satisfaction and retention. Achieving product/market fit is considered essential for the success of a startup or new product.
Question Bursts: For more info on the benefits of question bursts, check out: https://mitsloan.mit.edu/ideas-made-to-matter/heres-how-question-bursts-make-better-brainstorms
Jobs To Be Done: A theory and methodology for understanding customer motivations and needs in business and product development, based on the idea that customers "hire" products or services to fulfill specific jobs.
Investment Thesis: A clear, definable idea or set of ideas that outlines an investor's expectations and reasons for the potential outcome of an investment.
Exhaust data: refers to the data generated as a by-product of regular organizational activities and processes. This data can sometimes be repurposed or sold, offering potential additional value or revenue streams.
Geospatial data: information that has a geographical component, such as coordinates, addresses, or areas, related to points of interest and movements of people in and around the points of interest, is used in various analyses to spatially analyze trends.
Freedom of Information Act (FOIA) Requests: A legal process by which individuals or organizations can request access to government-held information, which must be released unless it falls under specific exemptions.
A/B Testing: a way to compare two versions of something to figure out which performs better. While it’s most often associated with websites and apps, the method is almost 100 years old and it’s one of the simplest forms of a randomized controlled experiment. https://hbr.org/2017/06/a-refresher-on-ab-testing