Is ChatGPT Getting worse? A Case Study on Confirmation Bias
Is ChatGPT-4 getting worse? You already know the answer you believe and if you don’t pause to catch the inherent biases associated with being human, you may miss what the evidence actually shows.
Welcome to the Data Score newsletter, your go-to source for insights into the world of data-driven decision-making. Whether you're an insight seeker, a unique data company, a software-as-a-service provider, or an investor, this newsletter is for you. I'm Jason DeRise, a seasoned expert in the field of alternative data insights. As one of the first 10 members of UBS Evidence Lab, I was at the forefront of pioneering new ways to generate actionable insights from data. Before that, I successfully built a sell-side equity research franchise based on proprietary data and non-consensus insights. Through my extensive experience as a purchaser and creator of data, I have gained a unique perspective that allows me to collaborate with end-users to generate meaningful insights.
How Is ChatGPT’s Behavior Changing over Time?
It’s an important question to answer. In a world where individuals are going to become more dependent on AI1 to assist with various tasks and decisions, it’s important that we have performance testing and improve the explainability2 of the models3.
MLOps4 professionals are at the front lines of this challenge and should be concerned about the performance of AI models because they know that the risk of enhancing machine learning5 and AI models is a risk of “catastrophic forgetting6,” where the base model is affected and no longer performs well. So, it's right to be vigilant about this risk and constantly test the model.
Furthermore, the explainability of machine learning and AI models is a major challenge for both the creators and users of AI-powered software. In particular for large language models (LLM)7, the nuance of the specific wording of the prompts and the order of prompts can result in different results even with the same intention. As more inputs are provided for the model, the unsupervised portion of the model may make connections differently than it has in the past. Furthermore, there is a variability setting in the model referred to as the “temperature8”, which allows for creativity in the answer, which makes it more human like.
So, I very much appreciate the work by Lingjiao Chen, Matei Zaharia, James Zou on “How Is ChatGPT’s Behavior Changing over Time?” https://arxiv.org/pdf/2307.09009.pdf
This is an important beginning of the process of improving the ability to independently test AI model performance and the ability to explain how the models work. I think a fair assessment of this paper is that it has moved the debate forward and raised new questions to answer. And, there’s more work to do.
However, many people jumped on the paper as proof that the model is performing worse. But let’s slow down and talk through what the paper actually said and what can actually be concluded from it.
I want to give credit to Arvind Narayanan and Sayash Kapoor who did a great job of breaking down potential improvements in how the model could be tested, building on what the paper has introduced. Here’s Arvind and Sayash’s thoughts on the study:
I also appreciate the authors making the effort to confirm their conclusions with Matei Zaharia, CTO of Databricks and Computer Science Professor at UC Berkley, who is a co-author on the paper. Here’s Matei Zaharia on Twitter, stating that they are not claiming there is an intentional degradation of the model.
https://twitter.com/matei_zaharia/status/1681515867803381762
“Yeah, definitely not claiming it’s cost saving or any other mechanism. It’s hard to tell why such changes happen, and it may be hard to monitor or control them.”
I think this is the most important aspect: “It’s hard to tell why such changes happen, and it may be hard to monitor or control them.”
My 2 cents on next steps as a way to help others think through similar diagnosis problems
A future study should consider establishing a suitable control group and incorporating tasks that fall within the typical capabilities of LLMs. We want to make sure that, as Arvind and Sayash point out, it's possible that the model from a language point of view is just answering yes always or no always.
Here's an example of a classic statistical conundrum: Let's say a machine learning model takes input from hundreds of variables to calculate the probability of a patient having a disease. It boasts an error rate of 1% and an accuracy of 99%. However, if the actual occurrence rate of the disease is only 0.5%, the model's accuracy would improve if it always predicted the disease's absence, regardless of the hundreds of variables.
The test used to measure model performance on prime numbers covered part of the story, but in many ways, it could just be finding that the model always predicted yes or no.
So we need precision and recall metrics9 to build a confusion matrix to understand the performance, which will help improve the study tests. Then calculate the F1 score10 of each model to compare performance. The higher the F1 score, the better.
If I had to state a hypothesis, I would suggest that ChatGPT is not good at passing prime number logic in any version because it’s a language model, not a math and logical reasoning model. For what it’s worth as an anecdote and not a scientific test, the latest Bard and Claude LLMs got the specific prime number test shown in the paper right, but when I started testing other numbers (both prime and not prime), it did get it right, but for the wrong reasons (one of them correctly said the odd number I provided was not prime, but because it was divisible by 2!). Also, GPT3.5 got it right and GPT4 got it wrong in my tests, but I also had the same inconsistencies with the other models when I gave it other numbers (both prime and not). A proper F1 test will reveal the above confusion matrix and a score that can be tracked over time.
I believe this approach, by setting up F1 tests applied to many more tasks, will provide a composite score to be devised to monitor model performance. The tests should be set up across a wide range of skills, including ones that the models should be good at (i.e., language-based tasks).
But what I really want to talk about is the mass reaction to the paper
Many people across the AI commentary landscape were very quick to jump on the paper as proof for their belief that the model is getting worse. Within 24 hours across multiple communities, we saw a huge number of comments saying across Twitter, LinkedIn, Reddit, Threads, etc., “I knew it was getting worse!” and specifically referring to the prime number test of the paper as proof.
What I liked about Arvind’s take is that he had a similar initial reaction on twitter that it was getting worse, but he revisited the paper and come out with a different conclusion.
“OK, I re-read the paper. I'm convinced that the degradations reported are somewhat peculiar to the authors' task selection and evaluation method and can easily result from fine tuning rather than intentional cost saving. I suspect this paper will be widely misinterpreted.” https://twitter.com/random_walker/status/1681508023083073537
This is the classic system 1 and system 2 thinking discussed by Daniel Kahneman in “Thinking, Fast and Slow” https://www.amazon.com/Thinking-Fast-Slow-Daniel-Kahneman/dp/0374533555 based on his decades of work with Amos Tversky.
We are humans, so we have biases.
There are many heuristics and biases in humans. Our minds are hardwired for rapid, automatic thinking, which evolved as a survival mechanism for our ancestors. This quick-response mode, known as System 1, is where cognitive biases take root.
System 2 is a slower, more thoughtful approach to problem solving. When we slow down and think through a problem, we get more accurate answers and decisions. However, for it to be really effective, we also need to be able to recognize that our initial assumptions and beliefs could be biased by the System 1 thinking.
Confirmation Bias
Wikipedia has a great definition: “Confirmation bias is the tendency to search for, interpret, favor, and recall information in a way that confirms or supports one's prior beliefs or values. People display this bias when they select information that supports their views, ignoring contrary information, or when they interpret ambiguous evidence as supporting their existing attitudes. The effect is strongest for desired outcomes, for emotionally charged issues, and for deeply entrenched beliefs. Confirmation bias cannot be eliminated, but it can be managed, for example, by education and training in critical thinking skills.” https://en.wikipedia.org/wiki/Confirmation_bias
People really wanted proof that the model was getting worse. What the paper tried to show was actually just how the model was changing in an attempt to understand the behavior of the model relative to its capabilities. But system 1 thinking said, “Yes! There it is! I’m right”. System 2 thinking said “let’s slow down and think about what the study is actually saying”.
I think we also have a bit of anchoring bias11 and the availability heuristic12 in this mix. As our social media feeds were already flooded with points of view that performance was getting worse and that the model was highlighted as performing poorly in a study by bright people wanting to add value to the debate as a first step, it reinforced the prior view that the model was performing worse and that most of what their feed showed was more support for that view.
Also, keep this bias in mind when you see a negative news headline about a famous person you don’t care for and immediately think, “I knew it!” at the negative news. Take a moment to dig in a bit deeper and consider counterfactuals that help us better understand cause and effect beyond the facts we see and beyond our prior beliefs.
I thought this infographic covered it well. Confirmation Bias lets only see the facts that confirm our beliefs instead of the full picture of objective facts, which could change our views.
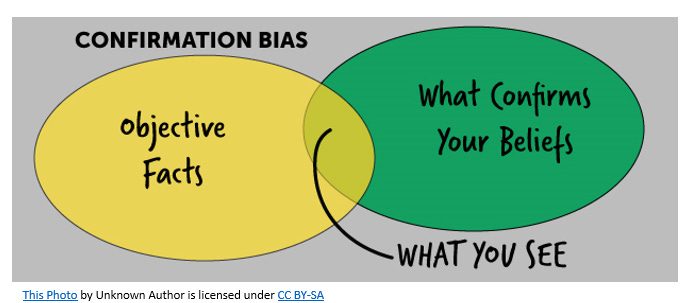
Confirmation Bias, Confirmation Bias Everywhere
A recent LinkedIn post by the author of the new best selling book “From Data to Profit” byVin Vashishta, pointed out the way people can also dismiss data points if they disprove a view by sharing a story of his daughter spending $400 on in app purchases, but potentially not being alerted by the bank. Borrowing this meme from Vin:
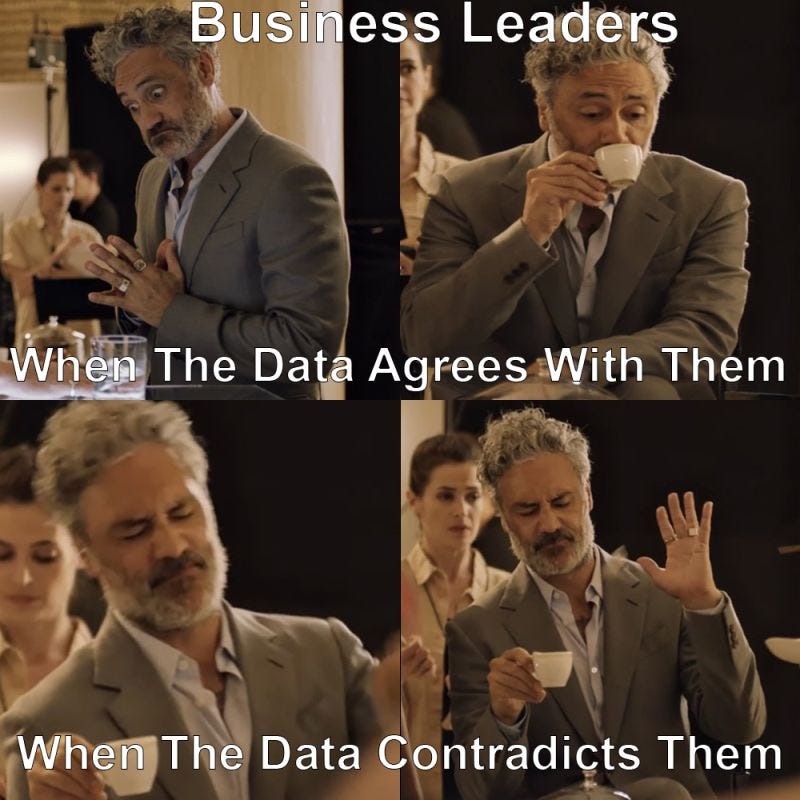
Marc Andreessen had a great quote on the impact of confirmation bias and how users of LLMs frame the problem of hallucinations on the Lex Fridman Podcast (at 18:13 minutes in).
Marc: There’s this issue of hallucinations and there’s a long conversation to have about that.
Lex: Hallucination is coming up with things that are totally not true but sound true.
Marc: Hallucinations is what we call it when we don’t like it and creativity is what we call it when we do like it. When the engineers talk about it they are like “Its terrible. It’s hallucinating”. If you [have] artistic inclinations, “Oh my god, we’ve invented creative machines for the first time in human history!”
#386 – Marc Andreessen: Future of the Internet, Technology, and AI**open.spotify.com
Me too- I have biases and I am not perfect in catching them
And I’m not on my high horse here, saying everyone else has confirmation bias, but I don’t. Anyone with access to the archive of UBS Sell Research can look at my published views at the beginning of 2014 and see how they played out, despite me having plenty of data that said I should have changed my views.
If you do take the time to find those, please check out my research accuracy in other years too please :)
In a future post, I’ll tell that story about how I had the data and the answers but fell into a number of heuristic traps. It’s something I’ve shared openly on stage in talks in the past. It was a humbling experience that changed my approach to taking in data points and changing how I think. Also, getting to work with some of the best analysts in the financial community showed me what good looks like when faced with data points that disprove their original view
But I’m not perfect at this. I welcome others to point out when I’m potentially falling into one of the traps of system 1 thinking when the situation actually calls for the more measured approach of system 2 thinking. Being aware is the first step in dealing with our own biases that come with being human.
Feel free to share your stories where our human biases get in the way of more advanced thinking, or feel free to share your thoughts on how we can test AI model performance.
- Jason DeRise, CFA
Artificial Intelligence (AI): The simulation of human intelligence processes by machines, especially computer systems, which include learning, reasoning, problem-solving, perception, and language understanding.
Explainability: The extent to which the internal mechanics of a machine or deep learning model can be explained in human terms.
Model: In the context of machine learning, a model is a representation of what a machine learning system has learned from its training data. Training is the process of teaching a machine learning model to make predictions by providing it with data.
MLOps or Machine Learning Operations: a practice for collaboration and communication between data scientists and operations professionals to help manage the production machine learning (or deep learning) lifecycle. It aims to shorten the development cycle of machine learning systems, provide high-quality and reliable delivery, and innovate based on continuous feedback and monitoring.
Machine Learning (ML): An application of AI that provides systems with the ability to automatically learn and improve from experience without being explicitly programmed.
Catastrophic Forgetting: A phenomenon in machine learning where a model, after being trained on new tasks, completely forgets the old tasks it was trained on. This is a significant issue in neural networks and an ongoing area of research in the development of AI models that can retain knowledge from previous tasks while learning new ones.
Neural Networks: A subset of machine learning, neural networks (also known as artificial neural networks) are computing systems inspired by the human brain's network of neurons. They're designed to 'learn' from numerical data.
Large Language Models (LLMs): These are advanced machine learning models trained on a vast amount of text data. They can generate coherent and contextually relevant sentences by predicting the probability of a word given the preceding words.
Temperature: a setting in AI that adjusts how sure or unsure it is when making guesses at the right answer based on probabilities of being seen favorably by the user. High temperatures make AI guess with more variability; low temperatures make it more confident and predictable.
Precision and Recall: Precision is the fraction of relevant instances among the retrieved instances, while recall is the fraction of the total amount of relevant instances that were actually retrieved. Both are used to measure the quality of a machine learning model.
F1 Score: The harmonic mean of precision and recall. It is used as a measure of a test's accuracy.
Anchoring Bias: A cognitive bias that involves relying too heavily on the first piece of information encountered (the "anchor") when making decisions.
Availability Heuristic: A mental shortcut that relies on immediate examples that come to mind when evaluating a specific topic, concept, method or decision.