Part 2: What goes wrong when business, finance, data, and technology worlds collide?
A 3-Part Series: Thriving Across the Worlds of Finance, Business, Data and Technology
Welcome to the Data Score newsletter, your go-to source for insights into the world of data-driven decision-making. Whether you're an insight seeker, a unique data company, a software-as-a-service provider, or an investor, this newsletter is for you. I'm Jason DeRise, a seasoned expert in the field of alternative data insights. As one of the first 10 members of UBS Evidence Lab, I was at the forefront of pioneering new ways to generate actionable insights from data. Before that, I successfully built a sell-side equity research franchise based on proprietary data and non-consensus insights. Through my extensive experience as a purchaser and creator of data, I have gained a unique perspective that allows me to collaborate with end-users to generate meaningful insights.
In part 1 we introduced this multi-part entry in The Data Score newsletter. We’re going to explore how to break down the silos between data, technology, and business to more easily get to the good part of a data insights practice: actionable, accurate decisions.
Part 1: Framing the problem: why silos are not viable anymore
Original silos and problems to be solved are mostly self-contained.
Problem complexity is accelerating.
Part 2: What goes wrong when the worlds collide?
Talking past each other
Smartest person in the room syndrome
Fear of failure
Part 3: How to break down the silos and align around outcomes
Build empathy.
Create proactive rituals.
Top-down culture
What I hope to offer here is a benchmark of the ideal for setting up the best possible outcomes when the worlds of business and finance come together with the worlds of technology and data.
I’ve seen the good, the bad, and the ugly in my experience working across all these worlds. But I want to be super clear on this: I don’t have all the answers. As I said in Part 1, I’m human, so I make mistakes too. I can’t promise that I’ve been perfect at following these ideals in the past. Nor can I promise that in the future there will not be times when communication will break down while I’m involved in the project.
The benchmark I put forward is to stimulate conversation. I’m keen to hear how everyone who reads this newsletter feels. Finance professionals, business leaders, data professionals, and technologists: how do you break down these silos to generate positive outcomes, even in difficult situations?
First, we diagnose the issues before building a better solution
Building bridges between the worlds of business, data, and technology to increase the overlap is critical to maximizing the return of a data and technology strategy for business decision makers. But first, let’s diagnose where the relationship goes wrong.
From my experience, the academic literature is right about organizations getting past 150 people and the pace of production slowing due to bureaucracy and organizational complexity. Even in organizations of 30 people or more, silos begin to form. And the majority of the Data Score readers work in or serve massive organizations with tens of thousands of employees who are trying to become more data- and tech-savvy in their approach to generating higher ROI.
Having one giant team is not possible, so we need to understand that functional and organizational silos are inherently going to happen in any organization that grows beyond the initial founding teams. Therefore, the challenge is in how the expertise from each silo comes together to achieve success.
The Analytics Power Hour podcast spoke about this subject of stakeholder buyer and creating alignment in the episode released on August 8th (shoutout to my friend Val Kroll, who joined the podcast as a regular host in this episode!).
The episode does really well to characterize what happens when business, data, and technology come together to make decisions that don’t go according to plan. They also offer suggestions to overcome it later in the episode, but I want to share a short section that highlights the struggle before I dig into how I would diagnose the underlying problems when experts across the different silos come together to problem-solve and make decisions.
Michael Helbling: I think that’s actually a really big one that it’s like the data is not gonna give you this perfect, definitive answer. I think it’s… I feel like it’s less if there is a definitive answer and it’s not what they wanna see, that they won’t accept it, I think just in general, that answers, the data provides always have a greater degree of uncertainty, they’ll look for a shred of uncertainty and say, well, but you’re saying you’re only pretty sure about this? Well, that’s my out to go do what I think because you need to give me the truth.
Tim Wilson: Yeah. Or the other side of it was sort of like, well, I was gonna do it anyway, so this is about the slimmest shred of evidence that I can find that gives me the justification for the thing I wanted.
Moe Kiss: Someone actually referred to it the other day as panning for metrics, and I was like, oh, that is a great term… I wanna be clear like this is obviously not every stakeholder.. these are intrinsic traits of being a human, of looking for information that you believe is true and things like that. It’s not like our stakeholders are real stupid or anything, I generally think they’re phenomenal people, but it’s like we’re trying to overcome the ways that our minds work, and that is even more difficult, I think, for someone that doesn’t specialize in data and understand the nuances with uncertainty and things like that.
Val Kroll: I think especially for more senior leaders who have been around the block and have some experience under their belt, they were making decisions long before they had access to this type of data, and so it’s a lot of unlearning of behaviors too. And so if they’re unable to model it, that’s like perpetuating that to their teams, and so it’s a lot of change management.
My view:
At the most basic level, when experts from business, finance, technology, and data come together around a single project with an expected outcome, there are inherent barriers to getting on the same page and aligning interests. I believe it’s the soft skills around communication, empathy, and psychological safety1 that make or break projects. Soft skills are needed to reach a win-win outcome even with well-documented requirements, project request forms, and data contracts in place to govern the initial deliverables and continued maintenance.
I believe the three most common reasons projects break down are:
Talking past each other
Smartest person in the room syndrome
Fear of failure
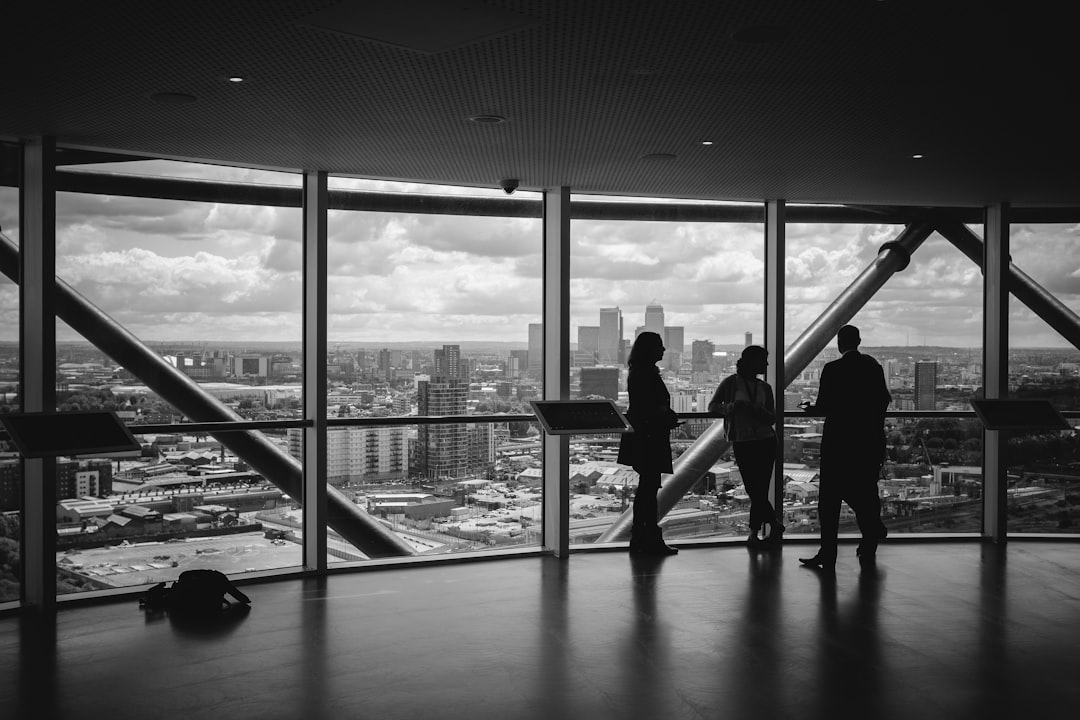
Talking past each other
The “how and why” each silo is making decisions often goes unstated because there’s an assumption that it’s already understood by the others. But that leaves the other side of the relationship without an understanding of the goals and blockers to success. Without that understanding, assumptions are made, which leads to misunderstandings about the priority and effort required.
Silos miscommunicate due to jargon and unstated motivations.
Jargon
The worlds of finance, tech, and data have an absurd amount of jargon. In the worlds of finance, data, and technology, the jargon used is helpful in distilling ideas down into a single word or phrase that captures a lot of meaning quickly. But it can also be a way that professionals can build barriers to entry by making their profession seem more complicated than it actually is.
Here are some abbreviations and jargon from both finance and data: P/E, DCF, LBO, SOTP, API, ETL, ELT, and RLHF2. Why would a data professional know what an LBO is? Why would a financial professional know the difference between ETL and ELT?
In actuality, the jobs of finance, data, and tech professionals are quite basic in logic and easily explained. It’s like a magician revealing the secret behind the trick; the actual logic is usually quite basic.
“Then you first learn how a magic trick is done, there’s often a moment of disappointment where you say, oh, he just held it in the other hand.” - Teller from Penn & Teller’s Masterclass on Magic
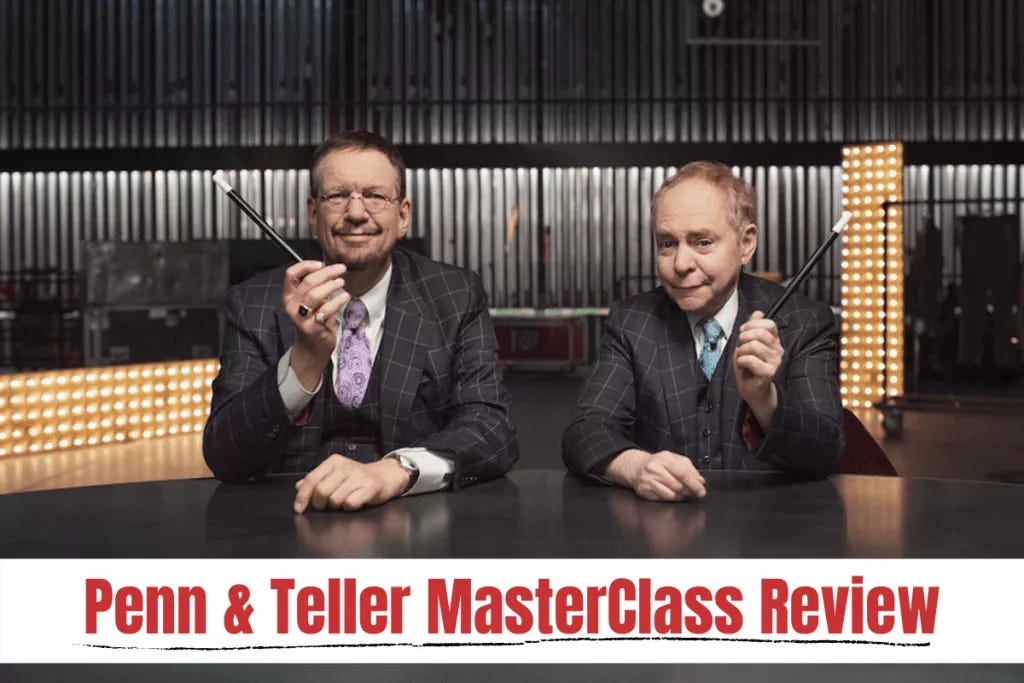
That’s why I’ve always included footnotes in The Data Score newsletter. Once the concepts are explained simply, none of them are hard to understand. But without a common language, it’s extremely hard to get on the same page.
Unstated motivations
The unstated motivations of each group become a blocker. The “how and why” each silo is making decisions often goes unstated because there’s an assumption that it’s already understood by the others. But that leaves the other side of the relationship without an understanding of the goals and blockers to success. Without that understanding, assumptions are made, which leads to misunderstandings about the priority and effort required.
Here’s a real example, without the details to protect those involved.
An analyst once asked for a forecast of what would happen to industry demand based on new capacity coming online in their sector based on different geographic demands and constraints. They were concerned with whether the new capacity would expand the market or cannibalize existing parts of the industry.
What the analyst was really asking for was a scenario analysis to explore what was possible. However, he didn’t say he wanted a scenario. He said he wanted a forecast.
But no single estimate was likely to be accurate over the long term because there are so many inputs that are constantly updated based on reality. There is a recursive nature to how companies in the industry would react to decisions about capacity made by their competitors and the consumer response, which in turn would drive companies to make more decisions.
The analyst really wanted to explore what was possible since the outcome would take years to play out and would likely take on many twists and turns along the way.
However, the request for a forecast was interpreted by the data/tech team as a request for a single-number forecast. And more so, this was going to be a great opportunity to build a cutting-edge machine learning model to provide a single, highly accurate estimate. The team wanted to go above and beyond what had been done before to show breakthroughs in predictive capabilities and ultimately generate more demand for similar types of analysis.
Unfortunately, when the data project was delivered, it was not accepted by the analyst. At first, he was happy with the initial estimate. But when he dug in deeper to understand the relationships between the variables and the output, his confidence began to fall. He asked, “What happens if demand from China increases?” The only appropriate answer was, “We can’t say without rerunning the model with new inputs.” At this point, it became clear that the need for scenarios and explainability was more important to the analysts than a single answer.
Unfortunately, the project did not progress from this point, with both sides disappointed in the outcome. Everyone did come to the project with the best intentions, but because unstated motivations were not made clear, it resulted in a failed project.
We’ll come back to this example in Part 3 of this series to see how it could have been avoided.
The smartest person in the room syndrome
Put three experts into a room with different expertise and all with the feeling that they are the smartest in the room, and it's highly likely that each will see the problem in their own way and not be able to get to the same page.
Apologies for the generalization I’m about to make, but in my experience, the worlds of finance, data, and technology tend to attract people to their industries who want to be seen as the smartest person in the room. Perhaps a reader of the Data Score with expertise in Psychology could help design a study to test my hypothesis.
The reality is that expertise across each of the worlds of business, finance, data, and technology requires specific training and practical experience. Therefore, no one can be the smartest person in the room when trying to solve a problem that requires all areas of expertise.
Put three experts into a room with different expertise and all with the feeling that they are the smartest in the room, and it's highly likely that each will see the problem in their own way and not be able to get to the same page. Even if the team is working together in planning and scoping meetings, the inability to step back and allow experts to offer views and shape the opinions of the other experts defeats the purpose of the meeting in the first place.
There is a heuristic3 that affects those who are experts in a space: they are so focused on why they are right that they miss why they can be wrong.
There’s been lots written on the concept, but this Atlantic article, “The Peculiar Blindness of Experts,” written by the author of the book “Range: Why Generalists Triumph in a Specialized World,” shares a great example of a bet between two experts on the impact of population growth and world hunger.
In the 1960s, Paul Ehrlich predicted widespread famine and starvation in the coming decades due to overpopulation. He felt the population was growing exponentially while the food supply was not.
Economist Julian Simon disagreed with Ehrlich's predictions. He felt human ingenuity and technology would increase the food supply and yield sustainable population growth.
The two made a famous bet in 1980 on whether commodity prices would rise (as Ehrlich expected due to scarcity) or fall over the next decade. Simon won the bet as prices dropped.
However, both men clung rigidly to their original positions, even in the face of evidence against them. Ehrlich claimed he was just slightly off on timing, while Simon ignored environmental warnings.
Each man focused only on information that confirmed his own view, becoming more dogmatic over time. Neither considered the valid points made by the other perspective nor used them to refine and improve their models.
The opposing experts were blinded by their confidence in their own expertise. Their narrow perspectives resulted in flawed predictions that could have been improved by integrating insights from other viewpoints.
Quoted from the article:
The catch: Commodity prices are a poor gauge of population effects, particularly over a single decade. The variable that both men were certain would vindicate their worldviews actually had little to do with those views. Prices waxed and waned with macroeconomic cycles.
Yet both men dug in. Each declared his faith in science and the undisputed primacy of facts. And each continued to miss the value of the other’s ideas. Ehrlich was wrong about the apocalypse, but right on aspects of environmental degradation. Simon was right about the influence of human ingenuity on food and energy supplies, but wrong in claiming that improvements in air and water quality validated his theories. Ironically, those improvements were bolstered through regulations pressed by Ehrlich and others.
Ideally, intellectual sparring partners “hone each other’s arguments so that they are sharper and better,” the Yale historian Paul Sabin wrote in The Bet. “The opposite happened with Paul Ehrlich and Julian Simon.” As each man amassed more information for his own view, each became more dogmatic, and the inadequacies in his model of the world grew ever more stark.
In summary, Ehrlich and Simon made poor predictions by failing to incorporate knowledge from outside their domains of expertise. An integrative approach considering diverse viewpoints may have yielded better forecasting.
The article also discusses Philip Tetlock and Barbara Meller’s work on prediction markets, which included the creation of two classifications of forecasters: hedgehogs and Foxes. From the article:
In Tetlock’s [and Meller’s] 20-year study, both the broad foxes and the narrow hedgehogs were quick to let a successful prediction reinforce their beliefs. But when an outcome took them by surprise, foxes were much more likely to adjust their ideas. Hedgehogs barely budged. Some made authoritative predictions that turned out to be wildly wrong—then updated their theories in the wrong direction. They became even more convinced of the original beliefs that had led them astray. The best forecasters, by contrast, view their own ideas as hypotheses in need of testing. If they make a bet and lose, they embrace the logic of a loss just as they would the reinforcement of a win.
Quotes and paraphrasing based on the full article found here: https://www.theatlantic.com/magazine/archive/2019/06/how-to-predict-the-future/588040/
Fear of failure
Financial markets feature high stakes, with pensioners and 401(k) holders relying on money managers to make sound decisions to secure enough capital to fund their retirement. Getting an investment wrong could be the difference between a 65-year-old teacher retiring this year or retiring many years later.
When the pressure is too high, research shows humans slip into system 1 thinking and follow heuristics that lead to suboptimal decisions.
System 1 and system 2 thinking discussed by Daniel Kahneman in “Thinking, Fast and Slow” https://www.amazon.com/Thinking-Fast-Slow-Daniel-Kahneman/dp/0374533555 based on his decades of work with Amos Tversky.
There are many heuristics and biases in humans. Our minds are hardwired for rapid, automatic thinking, which evolved as a survival mechanism for our ancestors. This quick-response mode, known as System 1, is where cognitive biases take root.
System 2 is a slower, more thoughtful approach to problem solving. When we slow down and think through a problem, we get more accurate answers and decisions. However, for it to be really effective, we also need to be able to recognize that our initial assumptions and beliefs could be biased by System 1 thinking.
When the pressure is on, thoughtful communication suffers
System 1 thinking is faster, automatic, and requires less effort. When time and mental bandwidth are scarce, System 1 thinking kicks in to provide quick responses.
High stress limits prefrontal cortex function; this impairs focus, working memory, and analytical reasoning associated with deliberate System 2 thinking.
Pressure focuses attention on immediate cues. System 1 thinking is oriented to the present moment and salient stimuli rather than the wider perspective of system 2 thinking.
Emotions triggered by pressure amplify System 1: feelings of stress, anxiety, and excitement all activate instinctive reactions.
Financial and business problems to be solved have high stakes.
Financial markets feature high stakes, with pensioners and 401(k) holders relying on money managers to make sound decisions to secure enough capital to fund their retirement. Getting an investment wrong could be the difference between a 65-year-old teacher retiring this year or retiring many years later. When you aggregate the impact of one client’s retirement with the same occurrence for many individual investors, the overall underperformance leads to assets under management being withdrawn by investors for better options.
To protect against this, an asset management company has risk management systems in place to monitor the performance of portfolio managers and assess if they should be given more capital to manage, less capital to manage, or even no capital to manage. This means there is more pressure and stress for the portfolio managers when faced with the challenges of an underperforming portfolio. Underperformance leads to the fear of falling into the latter bucket of being given no capital to manage.
And for data and technology professionals, there is increased stress too.
Let’s bring data and technology capabilities into the story now. As discussed in Part 1, the complexity and stakes associated with the problems to be solved are rising. Consider the stakes of the underperforming 401k compared to the impact of receiving a poor movie recommendation based on data science models.
In the case of the recommendation model failure, it just means I probably spend more time scrolling for a movie to watch.
In the case of the data science model failure for investing, it means retirement plans are affected, causing the individual to spend more time working and saving or reduce their lifestyle to meet their new lower retirement budget.
There’s more acceptance of errors in that data science problem compared to data science solutions that drive decisions about investments.
The models they build cannot possibly check every single data point or reflect scenarios outside of training data. There is always a fear that the model is wrong. I wrote about this risk in “The Paradox of Finding Surprising Insights in Alternative Data” https://thedatascore.substack.com/p/the-paradox-of-finding-surprising
As George Box is credited with saying, “All models are wrong, but some are useful.” Even knowing this as a data science professional, there’s still a risk that their work is not seen as useful by their finance and business stakeholders4. Or, more specifically, their own work and contributions are not useful and therefore are at risk of being let go.
Fear of being seen as suboptimal for problem solving or fear that business and financial decision makers will think they are better off using non-data-driven approaches can affect their own decision-making and communication.
As the pressure and challenge increase, the smartest people in the room, who are prone to talking past each other, cannot get on the same page about what to do next in the difficult situation they are presented with when things do not go to plan.
A quick word about fear of failure caused by impostor syndrome
As the pressure builds and expertise is needed across many realms beyond one’s core expertise, it’s common for anyone to feel like an impostor despite their strong expertise. From Wikipedia: https://en.wikipedia.org/wiki/Impostor_syndrome#cite_note-Langford19932-1
Impostor syndrome, also known as impostor phenomenon or impostorism, is a psychological occurrence in which people doubt their skills, talents, or accomplishments and have a persistent internalized fear of being exposed as frauds.
This also affects decision-making and collaboration when people feel this way. The important thing to know is that almost everyone feels this way, at least at some point. As Wanda Wallace said in her interview on The Corporate Bartender podcast: Well, I say if you don’t have the impostor syndrome, A, you’ve never pushed yourself to do something you haven’t mastered, or B, you’re obnoxiously arrogant.”
As someone who has felt this way many times in the past, I appreciate the direct approach to explaining the phenomenon that almost everyone has at some point felt this way. I think knowing this is liberating. If everyone feels this way, it’s normal if I feel this way sometimes too when working with other experts in their fields. As long as I still make the effort to try to add value and am open to other people’s views and open to recalibrating my own views, then this impostor syndrome-driven fear shouldn’t factor into my own behaviors as we work toward a solution.
Part 3, next week
In Part 3, I will offer solutions at an organizational level to help individuals and teams overcome the three core issues of talking past each other, the smartest person in the room syndrome, and the fear of failure. The solutions in Part 3 are based on my experiences seeing the good, the bad, and the ugly when the worlds of finance, business, data, and technology come together.
In the meantime, what other reasons do you believe cause the difficulties when data, technology, finance and business begin working closer together?
Links: Part 1 | Part 2 | Part 3
- Jason DeRise, CFA
Psychological safety: A culture where people feel comfortable taking risks and speaking up.
P/E (Price-to-Earnings ratio): A valuation measure that is calculated by dividing a company's share price by earnings per share.
Earnings per share is the net income of the company divided by the outstanding share count.
DCF (Discounted Cash Flow): A method for company valuation based on projected future cash flows.
LBO (Leveraged Buyout): An acquisition of a company using a significant amount of borrowed money to acquire a company, typically taking the company from the public market (with tradable shares on the stock exchange) to a privately owned company.
SOTP (Sum Of The Parts): a valuation approach that values a company by estimating the value of its divisions separately.
API (Application Programming Interface): Allows software programs to communicate with each other.
ETL (Extract, Transform, Load): Process for moving data from sources into a data warehouse and transforming the data before storing the transformed data on the server. This was the common approach when data was stored on physical servers, which limited the amount of data that could be saved.
ELT (Extract, Load, Transform): Similar to ETL, but in this process, raw data is loaded directly into the data warehouse. The transformation step happens later, whenever the data is needed. This is a more common approach when using cloud computing, which allows for data to be saved at a lower cost as well as the flexibility to expand the size of the storage.
RLHF (Reinforcement learning from human feedback): This is a machine learning model where the automated decisions are based on a reward-based scoring system where the various decisions the model can take are given rewards or penalties. The human is in the loop in the training process, where the direct feedback alters the future actions of the model.
Heuristic: an experience-based approach to problem solving that relies on rules of thumb.
Business Stakeholders is a term that is typically interchangeable with internal clients, but they should be considered more broadly to include all individuals in an organization affected by and interested in business outcomes.
Great write-up. Definitely see the smartest person in the room conflict arise more and more often.