Spreadsheets Are Dead. Long Live Spreadsheets.
From Finance to Formula 1: Exploring the use of excel while new technologies have evolved
Welcome to the Data Score newsletter, composed by DataChorus LLC. The newsletter is your go-to source for insights into the world of data-driven decision-making. Whether you're an insight seeker, a unique data company, a software-as-a-service provider, or an investor, this newsletter is for you. I'm Jason DeRise, a seasoned expert in the field of data-driven insights. As one of the first 10 members of UBS Evidence Lab, I was at the forefront of pioneering new ways to generate actionable insights from alternative data. Before that, I successfully built a sell-side equity research franchise based on proprietary data and non-consensus insights. After moving on from UBS Evidence Lab, I’ve remained active in the intersection of data, technology, and financial insights. Through my extensive experience as a purchaser and creator of data, I have gained a unique perspective, which I am sharing through the newsletter.
Despite repeated predictions of their demise, spreadsheets and Excel specifically remain the cornerstones of data analysis and business operations. While tools like Python for scripting, R for statistical analysis, SQL for database querying, and generative AI1 for advanced modeling are gaining traction, Excel's unique combination of accessibility, versatility, and integration means it’s not going away any time soon. Excel excels in scenarios requiring quick, user-friendly data manipulation and visualization, whereas more specialized tools are better suited for complex, large-scale data analysis tasks. However, as the complexity of problems to be solved increases, the amount of data needed to be wrangled and analyzed efficiently also increases.
Evolution and Adoption of New Technologies
Modern cloud databases offer scalable storage solutions and efficient compute resource allocation for data processing tasks. Utilizing SQL for querying, Python for scripting, and R for statistical analysis, along with advanced machine learning and AI tools such as TensorFlow, PyTorch, and Scikit-learn, enables deeper and more sophisticated analytics. These capabilities go beyond what traditional spreadsheet tools like Excel can provide, allowing for more comprehensive data insights and decision-making. The future of data analysis is more advanced, with many arguing that Excel has become outdated.
Despite the increasing integration of advanced technologies, spreadsheets still hold their ground. Excel’s adaptability and user-friendliness make it a staple in many professions, especially in finance and business operations. Spreadsheets offer a level of simplicity that appeals to the layman while also providing advanced functionalities for more tech-savvy individuals. Hence, while the expanse of data analysis tools continues to grow, the spreadsheet remains a resilient and valuable tool for many.
With the advance of new technologies, claims of the death of spreadsheets are a common cry. Let’s explore this in more detail.
Reflections on my Excel experience, back when it was cutting-edge for business analytics
I’m going to age myself. Back at Syracuse University, in spring 1999, I took a required undergrad class on business management (SOM 123) that included a lab on Excel—on Fridays at 8:30 am. Can you believe it was “so early!?” and on a Friday!? Little did I know that my life as a sell-side2 analyst would revolve around Excel. Little did I know that there was a point in time where I would dream in Excel (not something anyone should aspire to). And on that early class time, little did I know I would regularly arrive at the office before 7am each day, needing to be on my “A game” to digest and interpret the investment implications of breaking information within 10 to 15 minutes of the event, with risk that the wrong guidance could cost the firm millions.
In my twenties, Excel was a critical part of my job in finance. When I started as a junior analyst at Sanford Bernstein, I remembered little from that Syracuse course (apologies, Professor Diz). I was comfortable with Excel, largely due to using it for fantasy sports. But little did I know that I was still an amateur at Excel.
On my first day at Sanford Bernstein, the senior associate on my team was helping me get set up. She was an ex-Goldman investment banker, sharp in financial analysis within the healthcare sector, and a wiz at Excel. On my first day at Bernstein, she showed me my desk and helped me log in. Then she took the mouse and flung it off the desk, leaving it dangling from the desk by the chord. She then popped the F1 key3 off the keyboard and said, “Now you’re ready to work.”
The most productive associates at Bernstein were geniuses at Excel. They could not only perform the basics faster and gain more insights from data but also have time to think deeply about these insights, craft investment theses4, and visualize data to support their view.
I learned rapidly from others how to master Excel. I saw that they could rapidly get raw data into Excel and transform it into insights, all without touching the mouse. They used advanced formulas and analytical features to to wrangle and cleanse the data. I had multiple cheat sheets printed and pinned around my “Office Space"-style cubical and forced myself to get into the habit and muscle memory for Excel.
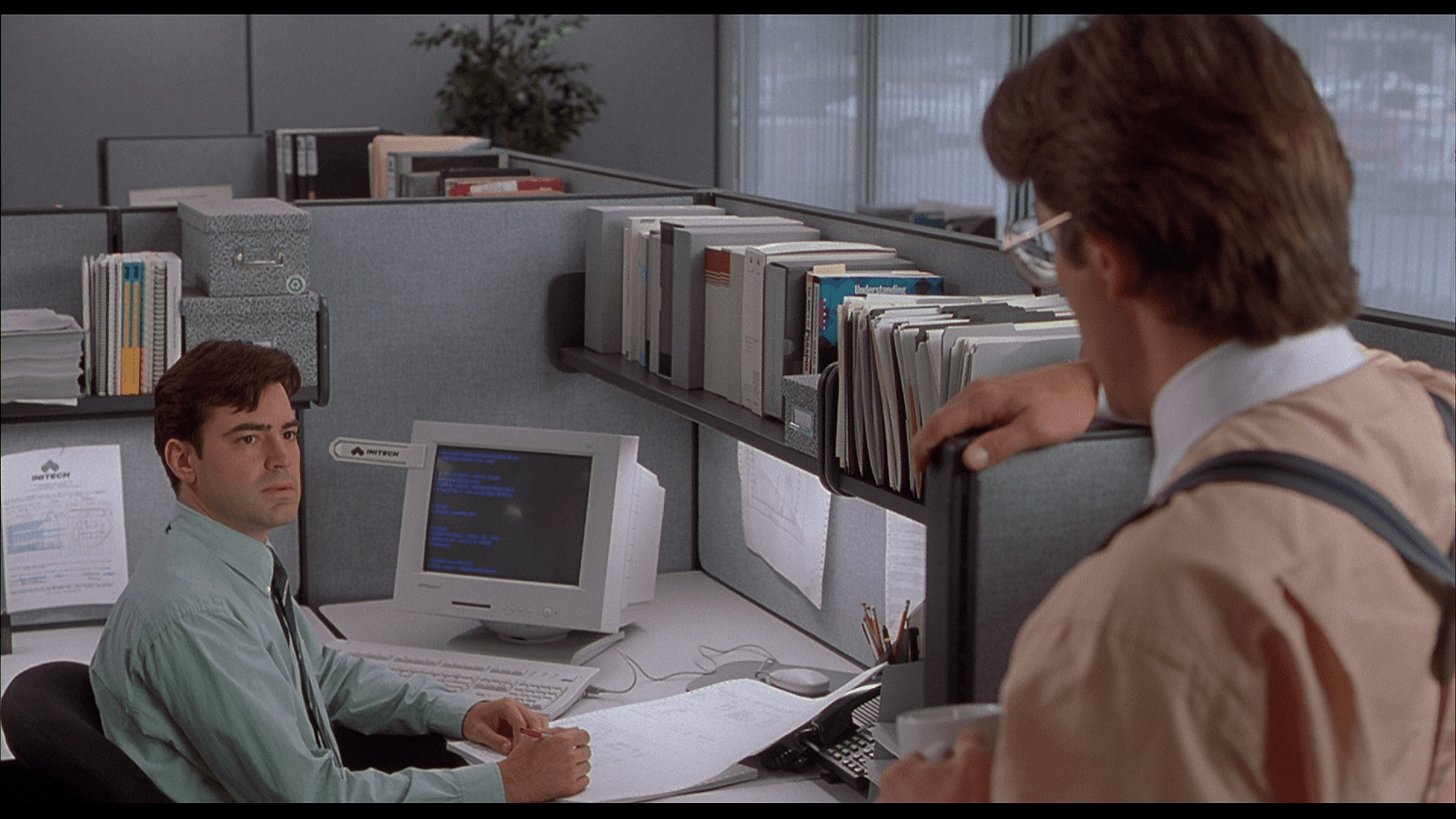
To me, it was like learning a language and an instrument. At some point, it was just intuitive. When people would ask me how good I was at Excel, my answer was, “Sometimes I have to touch the mouse.”
I also wanted to pay it forward when I saw some colleagues struggling with Excel, taking many steps to manually copy and transform data. As a contrast to the stories of Excel wizzes, I learned one of my colleagues was working for a senior analyst who would stand over her shoulder with a calculator, telling her what numbers to hard code into the financial model as they worked through the updates on earnings day. I guess the senior analyst missed the point that Excel is just a big calculator.
Beyond the core spreadsheet capability, Excel continued to advance with increased capabilities from the integrations of VBA5 and Microsoft Access. These tools allowed for some of the earliest use cases in financial markets, leveraging larger datasets sourced from the web to generate insights well before the term alternative data6 existed. On the Alternative Data Podcast in August 2023, Mark Flemming Williams wondered about my experience with Alt Data in the mid-2000s. Excel and Microsoft Access made it possible, even though it wasn't called Alt Data then.
Learning about the perils of over reliance on Excel from outside the financial markets
Looking outside the financial markets for examples where the industry has moved on and potential left slow adopters of new technology behind.
As an example of the derision for Excel, let’s look to another industry Instead of looking to the financial community: the world of F1 racing.
F1 Racing: Williams Racing’s dependency on Excel
In F1, there’s 10 teams with 2 star drivers and a thousand person teams, including technical expertise, data scientists, engineers, and rocket scientists to enable the cars and drivers to perform at the limits of physics to win races and capture share of prize money and advertising wallet to generate a return on investments.
As preparations began for the 2024 season, reports began to note that the Williams F1 racing team was behind their competitors in releasing their new car. But this story starts at the end of the 2022 season. Williams finished last place (10th). Williams hired a new team principle named James Vowles in January 2023, who had moved from the highly successful Mercedes team where he was Chief Strategist. The Mercedes team is known for its best-in-class data and analytics that help maximize the performance of its cars and drivers. The 2023 season showed improvement, with the team rising from last place in 2022 to 7th in 2023. Williams was expected to continue improving. However, the first race of 2024 was met with disappointment for the team.
The press uncovered in interviews with James Vowles his concern about how Williams operates behind the scenes, calling out the dependency on excel to build and maintain the car. From the article titled “THE SHOCKING DETAILS BEHIND AN F1 TEAM'S PAINFUL REVOLUTION,” which includes the subheading “A CAR BUILT IN EXCEL?!”
It is not an exaggeration to say that up to and including at least the initial work on the 2024 Williams, its car builds were handled using Microsoft Excel, with a list of around 20,000 individual components and parts. Unsurprisingly, ex-Mercedes man Vowles—someone used to class-leading operations and systems—had a damning verdict for that: “The Excel list was a joke. Impossible to navigate and impossible to update.”
Managing a car build is not just about listing all the components needed. There wasn't data on the cost of components, how long they took to build, how many were in the system to be built.
“Take a front wing,” says Vowles. “A front wing is about 400 different bits. And when you say I would like one front wing, what you need to kick off is the metallic bits and the carbon bits that make up that single front wing.
“You need to go into the system, and they need to be ordered. Is a front wing more important than a front wishbone in that circumstance? When do they go through, when is the inspection?
“When you start tracking now hundreds of 1000s of components through your organisation moving around, an Excel spreadsheet is useless.
“You need to know where each one of those independent components are, how long it will take before it's complete, how long it will take before it goes to inspection. If there's been any problems with inspections, whether it has to go back again.
“And once you start putting that level of complexity in which is where modern Formula 1 is, the Excel spreadsheet falls over, and humans fall over. And that's exactly where we are.
“There is more structure and system in our processes now. But they are nowhere near good enough. Nowhere near.”
Originally reported: https://www.the-race.com/formula-1/what-we-learned-bahrain-grand-prix-day-one-formula-1-2024/ and the quotes above via https://www.the-race.com/formula-1/shocking-details-behind-painful-williams-f1-revolution/
Before moving on, I’ve got to shout out Dan Entrup’s newsletter for sharing the meme below after the Williams news broke. The financial markets are heavily dependent on spreadsheets in Excel to make critical decisions.
Spreadsheets are dead. Long live spreadsheets.
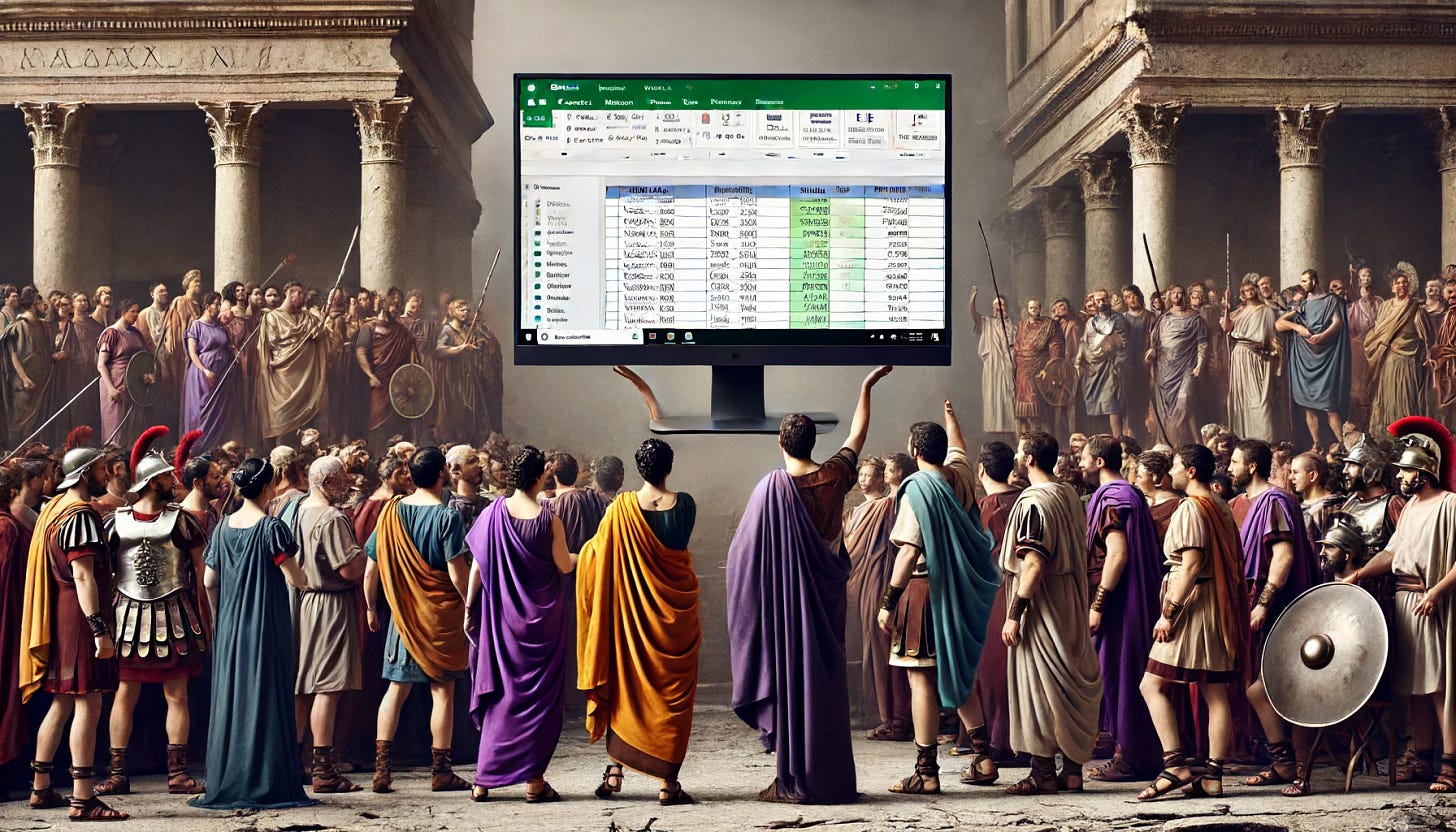
Are spreadsheets dead? In this modern age, I asked ChatGPT 4o to act as two experts, arguing the merits of spreadsheets continuing to be used vs. the merits of retiring spreadsheets. I then asked it to assess the winner of the arguments and score how important each topic is to the overall debate.
Here’s what ChatGPT provided.
Ironically, Substack still doesn’t support code markdowns within its publishing platform. So I had to take the table created, put it into, you guess it, Excel, and take a screen shot of it to include here.
ChatGPT Commentary: The importance scores reflect the weight each factor carries in the broader context of spreadsheet use in data analytics and their potential future with AI integration. This helps in evaluating the overall effectiveness and sustainability of spreadsheets as a tool in modern data-driven environments.
Logically, we should have moved on from spreadsheets, but it is unlikely to actually happen any time soon
Despite arguments for their obsolescence due to technological advances, spreadsheets remain the go-to tool for user analysis. Data products and services have developed numerous interfaces and experiences for exploring and consuming data-driven insights. Great data products provide trusted insights that answer users' economic questions, leaving them only to make decisions. These products reverse the 80% prep time, 20% analysis time paradigm. With 80% of time spent on analysis, better and faster decisions are possible. However, the additional time for thought rather than data wrangling will lead to new questions not directly answered by the data product. When that happens, the non-technical stakeholder’s most likely next step will be downloading data into Excel to figure out the answer.
We’ve all seen what it looks like. A download from one dashboard with important information is then combined with download data from another database with relevant information and maybe a third source. Of course, none of these downloaded datasets have a common joining key or an easy way to consolidate and aggregate them into new insights. So the user creates a complex series of tables, worksheets, and lookup formulas (with manual overwriting of cells that can’t be easily replicated for the next refresh) that are used to mix and match the data. We’re back to the 80% prep-time. And don’t forget about what happens when the user wants to refresh the analysis. All those manual steps need to be replicated, which leads to potential for errors. Plus, its common for the upstream data sources to change, requiring more manual patches to the series of excel sheets to maintain the analysis. This is what the Williams racing team’s situation sounds like.
Why do this when Python or SQL could handle it in just a few lines of code? This is primarily because the comfort level with Python, SQL, or other coding languages is low on the business side of companies. The users are data-savvy but not coding savvy. Spreadsheets bridge the gap quickly.
The adoption of Generative AI will also provide the ability for non-technical staff to create and debug code to wrangle data efficiently.
Like Excel has added Python to its product (in preview at the time of writing), one can imagine a Generative AI-driven co-pilot aiding the Excel user on how to wrangle the data across multiple sources, which may ultimately be done via Python, but within the spreadsheet.
But most financial professionals are not yet using these generative AI tools to try to learn how to incorporate coding into their workflow.
Newer generations and newer technologies
This isn’t a “Get off my lawn!” moment for The Data Score Newsletter. As technologies become available, newer generations of workers start their career comfortably leveraging them. Tableau, SQL, Python, R, and the plethora of foundational AI models available for use to turn raw data into insights. Also, Excel doesn’t have a monopoly on spreadsheets, as a new generation of analysts has grown up leveraging Google Sheets in their academic careers.
Technologies change and it is normal for once-cutting-edge capabilities to become obsolete. But this will take time to play out. As a new generation of workers enters with more advanced coding skills than prior generations, similar to prior generations leveraging the mainstream technology of the time, there will be a gradual shift from depending on spreadsheets for wrangling data. Excel replaced prior methods of analyzing and storing data and new employees who harnessed its power to make a difference got ahead of those who didn’t. The same will happen for newer technologies.
In the interim period, the complexity of the problems to be solved will continue to increase, requiring more data and advanced capabilities to be brought to the solution. Those with the technical ability to leverage more advanced capabilities still need to plan for the output to be small enough to fit into a spreadsheet if additional analysis by a non-technical stakeholder is needed.
Where do you stand? Spreadsheets are dead? Or, long live spreadsheets?
- Jason DeRise, CFA
Generative AI: AI models that can generate data like text, images, etc. For example, a generative AI model can write an article, paint a picture, or even compose music.
Sellside typically refers to investment banking and research firms that provide execution and advisory services (research reports, investment recommendations, and financial analyses) to institutional investors. Buyside typically refers to institutional investors (Hedge funds, mutual funds, etc.) who invest large amounts of capital.
The F1 key in Excel is the help button. The reason to remove the F1 key is that the F2 Key is how to enter the cell to audit and edit the cell. Escape lets you exit the cell. So the F1 is easily bumped, causing the help feature to pop up and slowing down the work.
Investment Thesis: A clear, definable idea or set of ideas that outlines an investor's expectations and reasons for the potential outcome of an investment.
VBA: Visual Basic for Applications. Programming language embedded in Microsoft applications like Excel.
Alternative data: Alternative data refers to data that is not traditional or conventional in the context of the finance and investing industries. Traditional data often includes factors like share prices, a company's earnings, valuation ratios, and other widely available financial data. Alternative data can include anything from transaction data, social media data, web traffic data, web mined data, satellite images, and more. This data is typically unstructured and requires more advanced data engineering and science skills to generate insights.