What Alt Data Should Learn from Alt Rock
Exploring the art and science of bringing alternative to the masses
Welcome to the Data Score newsletter, your go-to source for insights into the world of data-driven decision-making. Whether you're an insight seeker, a unique data company, a software-as-a-service provider, or an investor, this newsletter is for you. I'm Jason DeRise, a seasoned expert in the field of alternative data insights. As one of the first 10 members of UBS Evidence Lab, I was at the forefront of pioneering new ways to generate actionable insights from data. Before that, I successfully built a sell-side equity research franchise based on proprietary data and non-consensus insights. Through my extensive experience as a purchaser and creator of data, I have gained a unique perspective that allows me to collaborate with end-users to generate meaningful insights.
The rock and roll hall of fame band Foo Fighters recently released their latest, and very emotional, album after the surprising passing of the band's drummer, Taylor Hawkins, in March 2022. Unfortunately, this isn’t the first time lead singer Dave Grohl has lost a bandmate, as he famously was Nirvana’s drummer when frontman Kurt Cobain died by suicide in 1994. 28 years ago this month, Dave Grohl released the first Foo Fighters album, playing all the instruments on the record, which was extremely well received and paved the way for 11 total studio albums released to date.
This got me thinking about the origins of alternative rock and how it unexpectedly burst into the mainstream in 1991. There are parallels between alternative rock’s story and alternative data's story1, and there are some lessons to be learned.
Nirvana went from obscure to mainstream and changed the face of music for the next decade
Nirvana’s Nevermind album, led by their first megahit, “Smells Like Teen Spirit,” has been credited by many for marking the beginning of alternative rock’s mainstream journey.
I recall being an 11-year-old who at the time listened to music from New Kids on the Block, Vanilla Ice, and Bon Jovi, but when I saw the Nirvana Smell Like Teen Spirit video, I was just absolutely amazed by the whole concept. I couldn’t get enough of it. I had my father take me to the music store so I could buy the cassette tape. I picked up an electric guitar that I had once taken lessons for as a 9-year-old and asked for the sheet music to learn the songs on the guitar.
I wasn’t alone. Nirvana’s music videos aired constantly on MTV, and songs played on top 40 radio stations. Loud, extremely distorted guitars and screaming lyrics were being aired on top 40 pop radio? In January 1992, the album became the number one album on the billboard top 200 by passing Michael Jackson’s “Dangerous” album, signaling the next generation of music.
Before 1991, there was a growing alternative rock scene in multiple cities (not just Seattle, where Nirvana was from). But was outside the scope of mainstream consciousness. There was always an audience for the music, but something changed, and it became mainstream music.
There’s an analogy for the alternative data sector with the early days of 90’s alternative rock.
Early pioneers in the Alternative Rock scene, especially those grunge rock bands from Seattle, would lament the label “alternative rock”, noting “it’s just rock music”. Often, I find myself channeling 90s alternative rockers in claiming that alternative data is just data.
In addition to sharing the “alternative” label and the parallel journey into mainstream adoption, there are deeper learnings from exploring the analogy. Alternative Data companies want to be different from mainstream data. They want to stand out with something unique and make an impact. But they also want to be commercially viable and have broad adoption.
Exploring why Nirvana’s “Nevermind” album so well received by mainstream audiences
How did an alternative rock band like Nirvana become a mainstream music act? Perhaps it is, as Michael Stipe (singer of the alternative rock band R.E.M.) described it at Nirvana’s Rock and Roll Hall of Fame induction:
“It is the highest calling for an artist as well as the greatest possible privilege to capture a moment, to find the zeitgeist, to expose our struggles, our aspirations, our desires, to embrace and define their time. Nirvana captured lightning in a bottle.”
And while this is likely true and there is also an element of serendipity in being in the right place at the right time, there is more to the story that most people don’t know. Two outsiders, Butch Vig and Dave Grohl, influenced the sound of Nirvana, making it easier for a mainstream audience to appreciate Kurt Cobain’s art. It is how they captured the lightning and put it in the bottle by bringing some familiarity to their novel artistic ideas.
Kurt Cobain was the artist with a unique sound, but with pop sensibilities beneath the surface
The first Nirvana album, “Bleach”, had all the “lightning” Michael Stipe referred to. It clearly showed the artistic expression that would define the band’s sound. It was made before Nirvana was widely known. While there are plenty of grungy, punky, and noisy rock songs on the album, there’s a familiar pop song structure hiding within the songs. For example, “About a Girl” is very much a pop song heavily influenced by the way The Beatles arranged their music. But the pop influences are hidden under the heavily distorted tones and aggressive rhythms that would later be labeled grunge music.
Kurt Cobain’s songwriting thoughtfully combined harmony and melody in surprisingly complex ways.
For anyone who wants to geek out on music theory, there’s an awesome YouTube video that explains how great Kurt’s songwriting is on “Smells like Teen Spirit.” At 13 minutes into the video, Rick Beato plays the chorus of the song on piano, highlighting the complexity of how melody and harmony intersect to create complex chords (though each part on its own is quite simple).
Inherently, Nirvana’s songs have familiar pop concepts hidden within the noisy guitars and screaming lyrics. But listening to their first album, it’s not easy to find, but it’s there. That changed on the second album.
Butch Vig was the producer who captured the lightning in a bottle
Butch Vig was the producer of the Nevermind album. He was critical to making Nirvana’s art accessible to a wider audience. To be clear, what is special about the music was always there in the writing by Kurt Cobain. What Butch Vig did was capture what was special, but he did it in a way that would be accepted by a broader audience. Though it is worth noting that this was done with initial reluctance by the band, who wanted to keep the recording process simple with a sound that was less processed,
Double tracking
Kurt wanted the album to sound like their live shows and have a raw sound. Butch had to convince Kurt to use tried-and-true recording studio capabilities. For example, Butch double tracked the guitars and vocals, which means he took the original recording and layered on top of it another track of the same part by the same musician, which fills out the sound. He was able to convince Kurt to agree to this production technique.
Again, for anyone who wants to geek out on the details, here’s Butch Vig talking about how they got to the chorus vocal sound on the song “In Bloom”, where they added harmonies and double tracking on the vocals (52 seconds in is the key part).
Playing to a click track
While recording one of the songs, the band kept speeding up at the chorus, and it was affecting the feel of the song. Butch Vig asked Dave Grohl to play his drum part to a metronome, which is a device that makes a clicking sound exactly at a specified beats per minute or tempo. Dave Grohl later shared in a documentary that when he was asked to do this, it felt like his chest was ripped open and his heart was ripped out (re-enactment of the event below). The click track removes the creativity of what the rhythm and “feel” of the drums can be because it forces a more precise adherence to a steady beat. Plus, a drummer is naturally supposed to do this on their own. But he agreed, and the recording of the song benefited from it. If you prefer the haphazard speeding up and slowing down, you can always find the many live recordings of the song “Lithium” where this always seems to happen. It’s another example of a tried and true mainstream music technique that allowed for alt-rock lightning to be captured in a bottle.
Dave Grohl brought in mainstream influences from an unlikely source
Dave Grohl wasn’t the drummer on the first Nirvana album. Actually, he was the fifth Nirvana drummer. He got the job by responding to a classified ad and auditioning. Dave is a self-taught drummer. While he has the ability to lay down complex drum beats, he often talked about his role in Nirvana as not messing up the amazing songs. So he kept the drum parts simple and tight, always supporting what the song needed.
Furthermore, he borrowed influences from an unlikely source: Disco. Yes, the Nirvana anti-mainstream sound has disco drum beats in the background.
Here’s Dave telling the story behind his drumming to Pharrell Williams
If you’re a fan of alt-rock, go back and relisten to the Nevermind album. Once you hear the disco beat influence, you can’t unhear it. It’s everywhere in the Nevermind Album. The decision to play drums in this style serves the songs well and makes the art digestible to the audience. The music has the excitement of something different, but it is also held together by the familiarity of the drums.
Product/Market Fit is a journey from acceptance of the novel as the familiar
Nirvana’s and alternative rock’s success in the 1990s is actually a tale of product-market fit and is an analogy for the alternative data industry. And there is science behind the journey from alternative to mainstream.
There is science behind this phenomenon
For music in the early 1990s, the audience had an unmet need. Audiences had grown tired of the glossy pop and overproduced hairband rock of the 1980s. As Michael Stipe said, people wanted a “zeitgeist, to expose our struggles, our aspirations, and our desires, to embrace and define their time.” Because Nirvana took on aspects of what was familiar, it was possible for them to succeed. The pure creativity of Kurt Cobain was not for everyone. It was too far out of the realm of what was expected or understood by audiences. Dave Grohl and Butch Vig made it accessible for a wider audience and changed the face of music, tapping into an unmet need in music.
To understand why novel ideas are not quickly accepted, we can look to psychology, specifically the Wundt Curve, Named for 19th-century psychologist Wilhelm Wundt. In research from 1960 by D.E. Berlyne leveraging work by Wundt, the concept suggests that enjoyment peaks when there is moderate novelty balanced with sufficient familiarity. Too much novelty causes fear and anxiety. And too much familiarity drives boredom. This insight in itself has grown in acceptance in more modern times, being referenced in multiple scientific studies and commonly used in the worlds of marketing and design to drive product adoption.
The Wundt curve shows that enjoyment rises with familiarity but then fades and maybe even becomes negative if it’s too common. This is because there is a level of comfort with the familiar. However, if something becomes too familiar, it becomes less enjoyable.
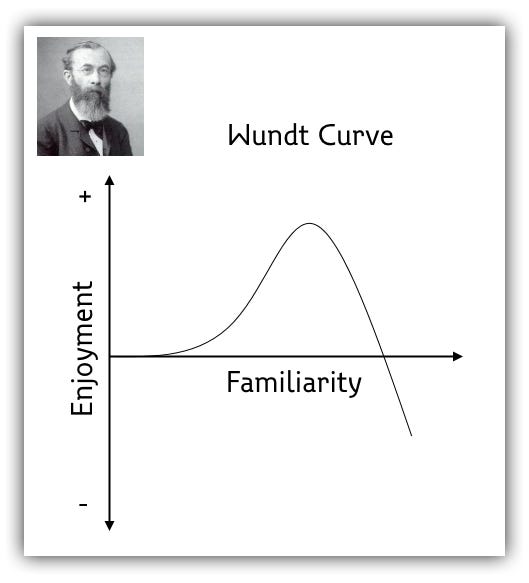
Alternative data needs to be better at merging the familiar with their novel products
In the context of investing, the novel approach has more potential for generating outsized alpha2 because it is not widely used. However, the novel will also face the highest levels of rejection and skepticism. The familiar approaches are where consumers are most comfortable.
But there’s a tipping point where the familiar and the novel intersect, and that’s when adoption accelerates.
This encapsulates the challenges faced by alternative data. Its novelty and unfamiliarity often push it beyond most investors' comfort zones. So how can alternative data providers walk Nirvana's line and blend progressive thinking with inclusive implementation?
The graphic below compares the Wundt curve to the potential impact of advanced thinking. There is more upside alpha potential from the use of earlier and novel approaches. However, the more novel the idea, the less likely it is to be adopted.
There are thousands of data companies with unique data and novel approaches to problem solving. But most are too far outside the familiar for their target audience in the financial community. While alternative data providers can pioneer data and technology artistry and garner a dedicated following, to reach commercial success, they need to align this creative approach with the proven methodologies of financial market analysis and scalable product development.
It’s important to note that in this framework of novel versus familiar, the level of peak familiarity could mean the data is available everywhere, but if it’s still explaining how the market is moving or is the consensus3, then there’s still value in the data. While the advancing thinking aspect is low, the ability to understand market movements (or beta4) is highly valuable and actually what data companies would aspire to. Effectively, at that stage, the data becomes part of the infrastructure of an insight platform. Perhaps in my music analogy, the comparison is to music that becomes a core part of culture, heard in movie soundtracks, background music at parties, part of cover band sets, etc.
Challenges in adopting alternative data as part of the core data stack
There are two primary blockers to wide adoption of new alternative datasets.
Most data companies misunderstand how financial market participants use data to make investment decisions
A significant portion of financial market participants struggle to extract actionable insights from data
These two blockers require additional effort to close the gap between the data products available in the market and the outcomes needed from the data by insight seekers. There’s limited time and resources to do the work to find insights from the data. The challenge of getting insights from data becomes harder when alternative data companies create products that are not aligned with the core “jobs to do” of their clients. The combination means good ideas for datasets cannot be converted to reality at the execution stage.
For more details on the challenges data companies have, check out this past entry in the Data Score: https://thedatascore.substack.com/p/why-some-data-companies-struggle
Some alternative data has already become mainstream
Mainstream adoption has been achieved before with data. For example, in the 2000s, as a consumer analyst, I was a high-power user of scanner transaction data5 to understand consumer behavior around consumer packaged goods purchases. It wasn’t called alternative data back then… it was just data.
In many ways, scanner data is core data for an investor in the consumer sector. Consumer transaction data sources have evolved over the years, but their adoption by investors has been high. The connection between revenue as a KPI6 of a consumer-facing business I care about and then understanding that the transaction data is a subset of that KPI is quite easy for investors, so the familiarity leads to easy adoption.
Even 5 to 10 years ago, there were alternative datasets that were on the periphery but are now considered core to a data insight stack. These include clickstream7, app analytics8, search data, and employee review data. Back in 2015, I spent my days helping analysts understand how these types of datasets could answer their critical questions, which were not easily answered by traditional data. There was plenty of skepticism then. Today, these datasets are very familiar and widely adopted by investors. This is the journey for data companies, as they begin at the fringe but grow in acceptance as familiarity increases.
Begin as niche, handcrafted, but build with scale in mind
It’s a journey that takes time and effort in the beginning, working with the early adopters to understand their needs and successes, and then amplifying the successes via social proofing (and generating some FOMO9) at the same time as building the product where the features that are “have to have” are scaled.
The idea of a handcrafted approach before finding market fit before scaling is covered here: https://thedatascore.substack.com/p/when-to-scale-or-keep-iterating\
In the early stages of product development, it's valuable to craft prototypes and explore potential uses, seek feedback from a niche audience, and then make choices that not only scale the product in terms of production but also foster wider client acceptance.
I am not suggesting that the data company remove or diminish what is special about the product. Instead, it’s about using tried-and-true methods that result in successful data products to make the product more familiar and accepted by a wider audience.
Examples include:
The product is easily joinable with traditional datasets
Standardized, easy-to-understand data schemas10
Symbology-enriched entities11
Point in time-stamped history12
Standardized metadata13
Standardized metrics
Data metrics mapped to traditional key performance indicators
Detailed support documentation
Transparency on revisions and data gaps
Flexible distribution and programmatic access
The data company also needs to build a narrative around the data product to cut through the noise and be memorable to clients. This requires:
A robust content strategy
An advisory service strategy
Think about where your data products land on a 2x2 grid of effort and impact in the eyes of the buyer. Data companies should be assessing the product from the point of view of their clients in terms of impact and effort. To engage asset managers beyond the top tier, data companies must align their products more closely with their clients' desired outcomes (impact) and ensure their data is easier to work with (effort).
Data companies can achieve success when they have unique and proprietary data that can generate a high impact but also make the product accessible and easy to use by adopting features that make the product more familiar to the users.
Like Nirvana’s adoption by mainstream audiences and prior generations of alternative datasets moving into the core data stack, there's a path forward for new alternative datasets to reach commercial success.
Have a product that is lighting: something special, proprietary, and valuable that meets the unmet needs of the audience.
Capture that lightning in a bottle by including features that improve familiarity to allow for wider adoption.
- Jason DeRise, CFA
Afterword
Improving resources for mental health is an important cause that I’ve donated time and resources to. With much of this article referring to Kurt Cobain’s positive impact before he took his own life, I wanted to suggest two causes to consider donating to that provide resources and support for mental health: the Jed Foundation (focused on youth mental health) and the National Alliance on Mental Illness of New York City (NAMI-NYC)
Alternative data: For anyone who’s stumbled on this article because of the Alternative Rock references, alternative data refers to data that is not traditional or conventional in the context of the finance and investing industries. Traditional data often includes factors like share prices, a company's earnings, valuation ratios, and other widely available financial data. Alternative data can include anything from transaction data, social media data, web traffic data, web mined data, satellite images, and more. This data is typically unstructured and requires more advanced data engineering and science skills to generate insights.
Alpha: A term used in finance to describe an investment strategy's ability to beat the market or generate excess returns. A simple way to think about alpha is that it’s a measure of the outperformance of a portfolio compared to a pre-defined benchmark for performance. Investopedia has a lot more detail https://www.investopedia.com/terms/a/alpha.asp
Consensus: “The consensus” is the average view of the sell-side for a specific financial measure. Typically, it refers to revenue or earnings per share (EPS), but it can be any financial measure. It is used as a benchmark for what is currently factored into the share price and for assessing if new results or news are better or worse than expected. However, it is important to know that sometimes there’s an unstated buyside consensus that is the better benchmark for expectations.
Beta: In finance, beta is a measure of investment portfolio risk. It represents the sensitivity of a portfolio's returns to changes in the market's returns. A beta of 1 means the investment's price will move with the market, while a beta less than 1 means the investment will be less volatile than the market.
In the context of the data, I’m referring to the data’s ability to explain the market’s movements because the data is widely available and therefore fully digested into the share price almost immediately. This level of market pricing efficiency means there’s not much alpha to be generated, but the data is still needed to understand why the market is moving.
Scanner transaction data: Information collected when a product's barcode is scanned at a point of sale. This data can be used to analyze consumer purchasing behavior, inventory management, and more.
Key Performance Indicators (KPIs): These are quantifiable measures used to evaluate the success of an organization, employee, etc. in meeting objectives for performance.
Clickstream data: This term refers to the recording of the parts of the screen a computer user clicks on while web browsing or using another software application. It can provide valuable information about user behavior and preferences.
App analytics: This refers to the measurement of user engagement and usage patterns within a mobile app. It can help identify how users interact with the app, what features are most used, and where users are facing issues.
FOMO: Fear of Missing Out
Data schema: In the context of databases, a data schema is an outline or a blueprint of how data is organized and accessed. It describes both the structure of the data and the relationships between data entities.
Symbology-enriched entities: In the context of financial data, symbology refers to a system of symbols used to identify particular securities (like stocks or bonds). Symbology-enriched entities would mean data records include these identifying symbols as metadata.
Point in time-stamped history: This phrase refers to a dataset that provides the time data to show how data has been revised. So it includes not only the time period the data was related to but also the date when the entire data set was originally released or revised. This allows investors to use the data in back-testing models as if it were seen in real time before revisions.
Metadata: Metadata is data that provides information about other data. In other words, it's data about data. It can be used to index, catalog, discover, and retrieve data.
Really enjoyed this. It made me wonder about rival goods: alt data is famously so, as every user is eating the others’ alpha. It feels like rock music can go either way: pop stars like Taylor Swift have economies of scale (part of the product is being in an arena with thousands of others whose parents paid thousands of $) while truly edgy and cool alt rock like early grunge is a rival good, and you’re supposed to move on to the next band when the first one gets too popular, at least if you’re trying to max your hipster alpha.