Will the data industry continue to consolidate?
Is the data industry heading towards more consolidation, M&A, and partnerships? Let’s apply analytical frameworks to frame the debate.
Welcome to the Data Score newsletter, composed by DataChorus LLC. The newsletter is your go-to source for insights into the world of data-driven decision-making. Whether you're an insight seeker, a unique data company, a software-as-a-service provider, or an investor, this newsletter is for you. I'm Jason DeRise, a seasoned expert in the field of data-driven insights. As one of the first 10 members of UBS Evidence Lab, I was at the forefront of pioneering new ways to generate actionable insights from alternative data. Before that, I successfully built a sell-side equity research franchise based on proprietary data and non-consensus insights. After moving on from UBS Evidence Lab, I’ve remained active in the intersection of data, technology, and financial insights. Through my extensive experience as a purchaser and creator of data, I have gained a unique perspective, which I am sharing through the newsletter.
In this edition, we explore whether M&A activity will continue in the data sector. Using a high-level analytic approach I often used as a sell-side analyst, we'll understand market dynamics and test a consolidation thesis using alternative data1.
Recent quarters have witnessed several high-profile mergers, acquisitions, and partnerships in the data industry. Consolidation has included combinations of data offerings in the same data domain, combinations of data offerings across different but related domains, large organizations acquiring niche capabilities, and cross border partnerships. These developments have been covered by the press and also by data-focused newsletters. Here’s a few to leverage to monitor the situation:
Each week, Dan Entrup includes a list of corporate actions2 across the data industry in his newsletter, It’s Pronounced Data:
Alex Boden’s Newsletter Asymmetrix profile’s data companies and analysis of announced M&A activities.
Matt Ober’s Rollup Newsletter provides his insights on recent M&A activity and thoughts about what could happen next. https://www.mattober.co/p/make-acquisitions
We also need to note there have been market exits with datasets no longer being offered as a form of consolidation.
Pick the right framework to assess industry structure
As a sellside analyst, my primary goal was to help the buyside3 make smarter investment decisions. To get to a useful view of what would happen next, it’s important to first understand the underlying drivers behind what was actually going on. Identifying key drivers and their interrelationships leads to better future modeling. I used various frameworks depending on the companies' situations. Some examples included:
Game Theory (like “The Prisoners Dilemma”)
Key drivers and constraints
“Whales and minnows”
Sigmoid curve (S-curve) analytics4
“Demographics is destiny” analytics
Scenario probability of non-linear relationships
Hidden valuation
Porter’s Five Forces
If there’s interest, we can do deep dives on each of these as it relates to the data industry. For the task at hand, we will use Porter’s Five Forces.
Porter’s Five Forces… but with an adjustment
Among these frameworks, Porter’s Five Forces is particularly useful for understanding industry dynamics. It breaks down the key factors affecting industry consolidation, making it ideal for our analysis. If one can understand the current industry dynamics, we can then assess the potential for changes in the structure of the industry.
Porter’s Five Forces is a framework for analyzing the competitive forces within an industry, assessing the intensity of competition, and identifying the potential for profitability. The five forces are: competitive rivalry, the bargaining power of buyers, the bargaining power of suppliers, the threat of new entrants, and the threat of substitute products or services.
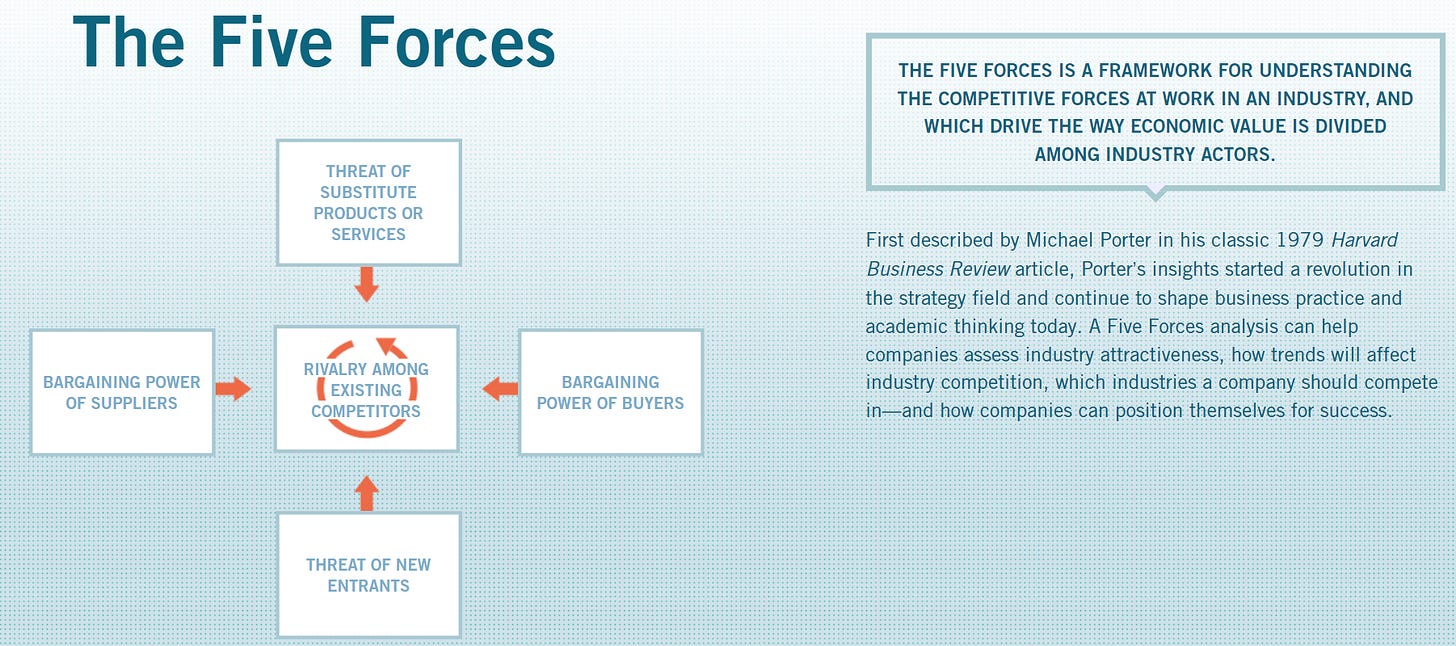
However, I would adapt the Five Forces model somewhat. The two primary factors that weigh into the model are the bargaining power of buyers and suppliers. Substitute products, new entrants, and competitive forces all weigh on the bargaining power of buyers and suppliers.
In addition, we need to add external factors to the framework, as regulation and government policies could shift the balance of power. Dramatic changes in technology can also have this disruptive power, turning industries on their heads. Porter’s Five Forces is more like a balancing scale, with external factors acting as “Lady Justice.”
Source: Dall-E… normally I post the prompt. But in this case I spent too much time and way too many prompts to get to this point. I could not figure out how to get the machine to understand the scales should not be balanced and represent the weights of buyers and sellers bargaining power. I especially enjoyed the program telling me it did as asked even though it clearly did not. We know Dall-E can’t spell so this is about as good as it gets. Maybe I can find an artist who’s willing to partner on some of the image ideas I have instead of using the machine.
Applying an adjusted Porter's Five Forces framework to the Data Industry
So let’s dive in to weigh customer purchase power versus supplier pricing power, using the other three forces as factors that can tip the scale, and consider external factors that ultimately calibrate the balance.
Background context
In some of the earliest Data Score posts, I highlighted my view that the data industry is currently in the trough of dissolution and explained why some data companies struggle to sell to the buyside. These provide more context for the summary provided below.
Data customer purchasing power:
Data budgets and the capacity to process large data sets follow a decay curve. There are asset managers who have the budgeting and technical capacity to purchase a wide variety of datasets, focusing on ROI5 rather than just price. They have what it takes to work hard with datasets and turn them into returns. But the majority of asset managers need to be more selective about which datasets they purchase. Or, more accurately, which investment debates they choose to address with data. They have to optimize their budget around economies of scope6.
Due to asymmetric information, buyers have strong purchasing power since the true value of data is often hidden from suppliers, unlike other B2B SaaS or DaaS products.
Switching costs are relatively low. The ability to switch between datasets has been a skill to survive in extracting insights from alternative data. Alternative datasets are inherently messy and there are times when datasets become unavailable for periods of time due to various issues; in some cases, the datasets go completely away. Being able to adapt to a changing environment is a required skill of the buyside using alternative data.
Threat of substitutes: Most market participants view substitute data products by domains, such as transaction data, mobility data, or clickstream data. However, data set types are not the right market segmentation. Data substitutes are defined by the questions the data sets can answer. Many paths lead to insights, depending on the market questions. And, those critical questions are constantly changing. So data can be substituted across data domains.
Each buyside firm has a unique combination of data sourcing within their repertoire of data to drive insights.
There is definitely overlap among suppliers that asset managers use in their insight stack. Some types of alternative data are more “core” than “alternative” and widely leveraged.
However, each asset manager can choose the best option for their investment strategy, drawing from the breadth of data options available and the nuances of understanding the key investment questions to answer. Effectively, each buyer of data is creating their own consolidation of data sources combined in their own environment to meet their needs.
Importantly, the cost of mixing and matching datasets in-house is not trivial, as datasets are not easily joined out of the box.
Potential for new entrants: There are low barriers to entry. New vendors are added to the market each month. The amount of exhaust data7 being made available to the market as an effort to monetize data assets trapped inside the corporate world continues to grow.
The cost to store data is falling, so it enables the easy availability of exhaust data converted to insight-ready data.
While it is easy to enter the market, the ability to stay is much different. Converting raw data into insights is a high-fixed-cost activity.
Notably, if a data vendor offers a truly proprietary dataset, it can favor the disruptive new supplier. But this disruptive entrant will win at the expense of, and the pricing power of, the incumbents. This does not support the pricing power of the entire supply side. If anything, others will copy the innovation and close the differentiation advantage. Such is capitalism.
Competitive rivals: The intensity of competition in the data sector, especially in selling to financial markets, is quite high.
Competition includes large data organizations and niche data companies specializing in specific sectors, geographies and consumer/corporate behaviors.
Within specific data domains, there are companies offering similar datasets.
Many data companies operate on a high fixed-cost model, making operating leverage8 a key profitability driver. Established players with both economies of scope and economies of scale9 are justified in competing on price to leverage their scale. Scale is a sustaining competitive advantage that shifts power to the suppliers.
Data supplier pricing power
OK, that’s a lot on the buyer's purchasing power. What about the levers the suppliers have?
The expanding supply in each data vertical limits pricing power.
The cost of scaling data products across multiple client personas is rising. Datasets with alpha10 potential can land with the most advanced data analytics asset managers with the largest data teams, even if the data is hard to use. But, to sell the rest of the market, the data provider needs to close the gap with more effort to turn raw data into easy-to-consume insights.
There are barriers to a profitable, scaled, data product primarily due to the human capital needed to traverse the intersection of data, technology, finance and business decisions. This is the trade off for the market. Does the provider take on the cost to make it easier to get insights or leave that to its customers? The latter may only work for a few buyers. The rest may know they could benefit from the insights, but only if they had the resource and ability to convert data into insight. In the absence of that ability, there is not ROI from purchasing new datasets.
Could technology tip the scales and allow anyone to get insights, making proprietary data more important than ease of use?
External factors: Technology & regulation could tip the data scales in the future
Can current and future market demand support over 3,000 data providers?
A compelling view was shared by Mark Flemming-Williams, who moderated a panel at Neudata’s recent conference. He highlighted an insight attributed to Abraham Thomas: that the growth in available affordable storage drove the ability for data to succeed, but there wasn’t enough demand for the supply. However, with the advance of GPU-powered11 compute and the acceleration of AI applications, the demand for data will be exponential. Could that tip the industry toward positive pricing power?
Conversely, the panel discussed current pricing pressure due to excess supply versus demand, with some competitors opting to compete on price. One potential from the panel discussion was that the cost of storage would fall and the ease of converting raw data to insights would fall, which would allow for more insights to be consumed, but at a lower cost per insight.
Economic and market performance may fuel or constrain the buyside depending on overall market performance.
Fund performance can affect data budget but these cyclical factors should not be confused with the underlying purchasing power of the buyside (market beta12). The market dynamics can be a near term external factor. This needs to be separated from the alpha generation of the specific datasets, which would determine if the datasets are generating ROI.
Market valuations can also impact specific valuations of data companies and that can sway the trend for consolidation. High valuations would deter activity.
Access to funding is also an external factor largly related to the economy and the market. Access to funding for growth initiatives can keep data companies growing on their own path without M&A. However, if that capital is unavailable, capital for M&A activity may be needed. And a lack of capital could lead to market exists through businesses closing their doors.
Regulation can tip the scales. These are known-unknowns… maybe unknown-unknowns.
As mergers and partnerships continue does the governement continue to look at the larger market definition or begin to look at specific dataset types?
Does regulation change around Data Protection Laws13 like GDPR rand rules around PII14, and other protections mean market participants need to exit the market. Do sources of raw data decide not to monetize their data?
Does AI regulation change the ability to process and generate insights at scale?
Thoughts on the outcome of the analysis
The scales tip toward buyers' bargaining power. However, certain factors still support pricing power for specific data companies
Data providers that have provable alpha generation
Data providers that have become the source beta15 (meaning everyone has access to the data because the information is explaining market movements and not having access to the data would hurt the ability to understand what’s moving security prices).
Data providers that have unique information that answers questions others cannot answer
Data providers who have multiple datasets that are easily combined and packaged for the buyer and are available to efficiently answer any investment question. The scale of the provider allows for more efficient conversion of raw data into insights.
The probability of more consolidation activity in the data provider space is more likely than not (which can be M&A-driven, partnership-driven, or from competitors exiting the market).
How might we test a thesis on the future of data industry consolidation with data?
How can we track if the consolidation thesis in the data industry is playing out? Follow the data: Here’s a brainstorming session on using alternative data sources to monitor the industry.
Consolidation data: monitor corporate actions with various data sources, including public and private markets. Sources can include Bloomberg, Refinitiv (owned by LSEG), Pitchbook (owned by Morningstar), and Crunchbase.
Rationale: Continued or accelerated corporate action across the industry would support more consolidation. Monitor frequency, breadth and size of activity to understand trends.
Monitor the popularity of data products through clickstream analysis, search activity, and B2B monitoring.
Rationale: interest in and usage of data products could reveal popularity of the offerings and if demand trends are shifting to support the current supply of data.
Monitor frequency and sentiment of press and earnings calls on data products
Rationale: the frequency and sentiment of data based on NLP16 techniques and financial markets would signal the overall mood of the industry and potential consolidation of sentiment is deteriorating. In addition monitoring the sentiment of the financial markets industry via earnings calls could reveal cyclical impacts on data purchasing budgets (eg is cost cutting a theme or growth a theme?)
Monitor supply of data via data platforms like Amass Insights, Eagle Alpha, Neudata and BattleFin
Rationale: if suppliers continue to increase without signals of data demand increasing, the less pricing power for the industry as a whole. Or, will supply consolidate firming the pricing power of the industry to the consolidated data offerings? Is the number of datasets available per provider increasing or are the number of providers with a 1 or 2 datasets growing?
Monitor assets under active management filtered by perspectives calling out investment strategies dependent on alternative data, systematic quantitative17 investing and advanced data analytics.
Rationale - the more assets under management for actively managed funds could support the structural need for a data driven investment process. I this can be further refined by filtering the data to funds who call out data driven insights as part of their investment strategy.
Monitor job postings at assets managers looking for data skill sets.
Rationale: the more active the buyside is in hiring data and tech professionals, the more demand is likely for data.
Monitor job postings at data companies. Monitor all jobs and especially sales people.
Rationale: more hiring by data companies equals more supply.
Monitor data patent applications, data patent ownership changes, data patent challenges
Rationale: the more new patent activity, the more likely new data supply is coming to market. However monitoring patent challenges and patent transfers could support a market consolidation thesis.
What other data sources could help monitor the state and structure of the Alternative data industry? Data companies feel free to comment below!
For more content like this, subscribe to the Data Score Newsletter, Composed by DataChorus LLC.
Feel free to share this with anyone who would also find this valuable.
- Jason DeRise, CFA
Alternative data: Alternative data refers to data that is not traditional or conventional in the context of the finance and investing industries. Traditional data often includes factors like share prices, a company's earnings, valuation ratios, and other widely available financial data. Alternative data can include anything from transaction data, social media data, web traffic data, web mined data, satellite images, and more. This data is typically unstructured and requires more advanced data engineering and science skills to generate insights.
Corporate Actions: Events initiated by a public company that bring changes to its securities, such as stock splits, dividends, mergers, and acquisitions.
Buyside typically refers to institutional investors (Hedge funds, mutual funds, etc.) who invest large amounts of capital, and Sellside typically refers to investment banking and research firms that provide execution and advisory services (research reports, investment recommendations, and financial analyses) to institutional investors.
Sigmoid function: a mathematical function having a characteristic "S"-shaped curve or sigmoid curve. S-curves are often used to describe the adoption of new technology.
ROI (Return on Investment): a performance measure used to evaluate the efficiency or profitability of an investment or compare the efficiency of a number of different investments. ROI tries to directly measure the amount of return on a particular investment, relative to the investment’s cost. https://www.investopedia.com/terms/r/returnoninvestment.asp
Economies of scope: cost advantages that a business obtains due to a broader scope of operations, often achieved by producing a variety of products or services using the same operations or resources.
Exhaust data: refers to the data generated as a by-product of regular organizational activities and processes. This data can sometimes be repurposed or sold, offering potential additional value or revenue streams.
Operating Leverage: One way to think about operating leverage is the sensitivty of operating profit growth (profit before interest and taxes) to changes in volume or revenue growth of the business. The primary driver of operating leverage is the mix of variable costs, which change as volumes change, and fixed costs, which do not change as volumes change. The more fixed costs as a portion of the business, the more sensitive operating profit is to changes in volume and revenue growth (declines).
Economies of Scale: Economies of scale are cost advantages reaped by companies when production becomes efficient. Companies can achieve economies of scale by increasing production and lowering costs. This happens because costs are spread over a larger number of goods. Costs can be both fixed and variable. https://www.investopedia.com/terms/e/economiesofscale.asp
Alpha: A term used in finance to describe an investment strategy's ability to beat the market or generate excess returns. A simple way to think about alpha is that it’s a measure of the outperformance of a portfolio compared to a pre-defined benchmark for performance. Investopedia has a lot more detail https://www.investopedia.com/terms/a/alpha.asp
GPUs: An acronym for "Graphics Processing Units." These are specialized electronic circuits designed to rapidly manipulate and alter memory to accelerate the creation of images in a frame buffer intended for output to a display device.
Beta: In finance, beta is a measure of investment portfolio risk. It represents the sensitivity of a portfolio's returns to changes in the market's returns. A beta of 1 means the investment's price will move with the market, while a beta less than 1 means the investment will be less volatile than the market.
Data Protection Laws: Legislation intended to protect individuals' personal data in the context of professional or commercial activity. An example is the GDPR (General Data Protection Regulation) in Europe.
Personal Identifiable Information (PII): Any information that can be used to identify an individual, such as a name, social security number, address, or phone number.
Beta In the context of data: In this case beta refers to the data’s ability to explain the market’s movements because the data is widely available and therefore fully digested into the share price almost immediately. This level of market pricing efficiency means there’s not much alpha to be generated, but the data is still needed to understand why the market is moving.
Natural Language Processing (NLP): An AI technology that allows computers to understand, interpret, and respond to human language in a quantitative way, generating statistical measures of sentiment and importance of topics.
Quant funds: Short for "quantitative funds," also referred to as systematic Funds. Systematic refers to a quantitative (quant) approach to portfolio allocation based on advanced statistical models, and machine learning (with varying degrees of human involvement “in the loop” or “on the loop” managing the programmatic decision making).