Top Questions ahead of Battlefin’s Discovery Day Miami
Battlefin is hosting their Discovery Day conference in Miami on January 24th–26th, 2024. Ahead of the conference, I've challenged ChatGPT to a competition: who can write the best questions?
Welcome to the Data Score newsletter, composed by DataChorus LLC. The newsletter is your go-to source for insights into the world of data-driven decision-making. Whether you're an insight seeker, a unique data company, a software-as-a-service provider, or an investor, this newsletter is for you. I'm Jason DeRise, a seasoned expert in the field of data-driven insights. As one of the first 10 members of UBS Evidence Lab, I was at the forefront of pioneering new ways to generate actionable insights from alternative data. Before that, I successfully built a sell-side equity research franchise based on proprietary data and non-consensus insights. After moving on from UBS Evidence Lab, I’ve remained active in the intersection of data, technology, and financial insights. Through my extensive experience as a purchaser and creator of data, I have gained a unique perspective, which I am sharing through the newsletter.
On January 24th through 26th, BattleFin is hosting its Discovery Day conference in Miami. https://www.battlefin.com/events/miami-2024
Ahead of the event, I’m sharing key questions on my mind for each speaker and panel.
Sponsored Advertisement
Flywheel Alternative Data showcasing collaboration with Arb Insights in Miami, including the new gross margin prediction tool. Access their latest research here.
There are two broad themes addressed by the questions proposed in this article
Preparing for the integration of emerging technologies into the analytics process
Uncovering data driven insights about current investment debates
Testing a practical use case for ChatGPT
I thought I’d have some fun with this article. I used ChatGPT4 to exact the agenda from the website and put it into the format below, so I didn’t have to do the work. It needed some extra prompting to make sure it did it accurately.
First, I gave it some examples of what the format should look like, leveraging prior Data Score conference previews, so it understood the format.
After it showed me that it could do it for a few examples that I provided, my prompt was
“That's great. But now I have a new agenda for you to organize that way. It's a longer agenda, covering 3 days. Please think step-by-step as you convert each agenda into the same output structure as you did above. Here is the agenda” (and I provided it with the full agenda). Yes, I am polite when prompting LLMs. One thing I learned from Star Wars is that the good side is polite to their droids!
The prompt didn’t work. ChatGPT was hallucinating1 the descriptions for each session.
I then followed up with a new prompt:
“Thanks please revisit the output compared to the agenda. I'd like you to 100% accurately use the descriptions provided in the agenda while creating your output. Remember the output should be 100% factual and match the agenda. The task is about reorganizing the data to meet the specified format provided above.”
This time, success. It got it right. I checked manually to compare the output to the original agenda. And on top of that it fixed some grammatical and punctuation items. The agenda it created was missing just one speaker, which I thought was an error on ChatGPT’s part, but actually I could see it was an error in my copy and paste from the agenda into the prompt. I did have to add the block quote formatting. I think ChatGPT could have noticed the formatting in my prompt examples, since it did add the bullets and bold formatting without me asking it to. Or maybe it couldn’t read the formatting of the block quote. So, I accepted that I would do this faster than spend more time trying to re-engineer my prompt a few times.
Man vs Machine
Then I thought, let’s see what it can do from a creative point of view. I really like Marc Andreessen’s quote from the Lex Friedman’s podcast about Gen AI Hallucinations and creativity,“Hallucinations is what we call it when we don’t like it and creativity is what we call it when we do like it. When the engineers talk about it they are like “Its terrible. It’s hallucinating”. If you [have] artistic inclinations, “Oh my god, we’ve invented creative machines for the first time in human history!”
#386 – Marc Andreessen: Future of the Internet, Technology, and AI**open.spotify.com
So let’s test out this creative machine.
Prompt: What would be the most thought-provoking questions to ask each panel as it relates to investing and data driven insights. Go step by step through the agenda, research the speakers and companies, and offer one question that would be highly relevant and useful for the audience to hear the answer. Be sure to show the question next to the agenda items summarized above so its clear which panel should recieve which question.
I’ll show the ChatGPT question and my question— A less dramatic version of John Henry versus the Machine plays out below.
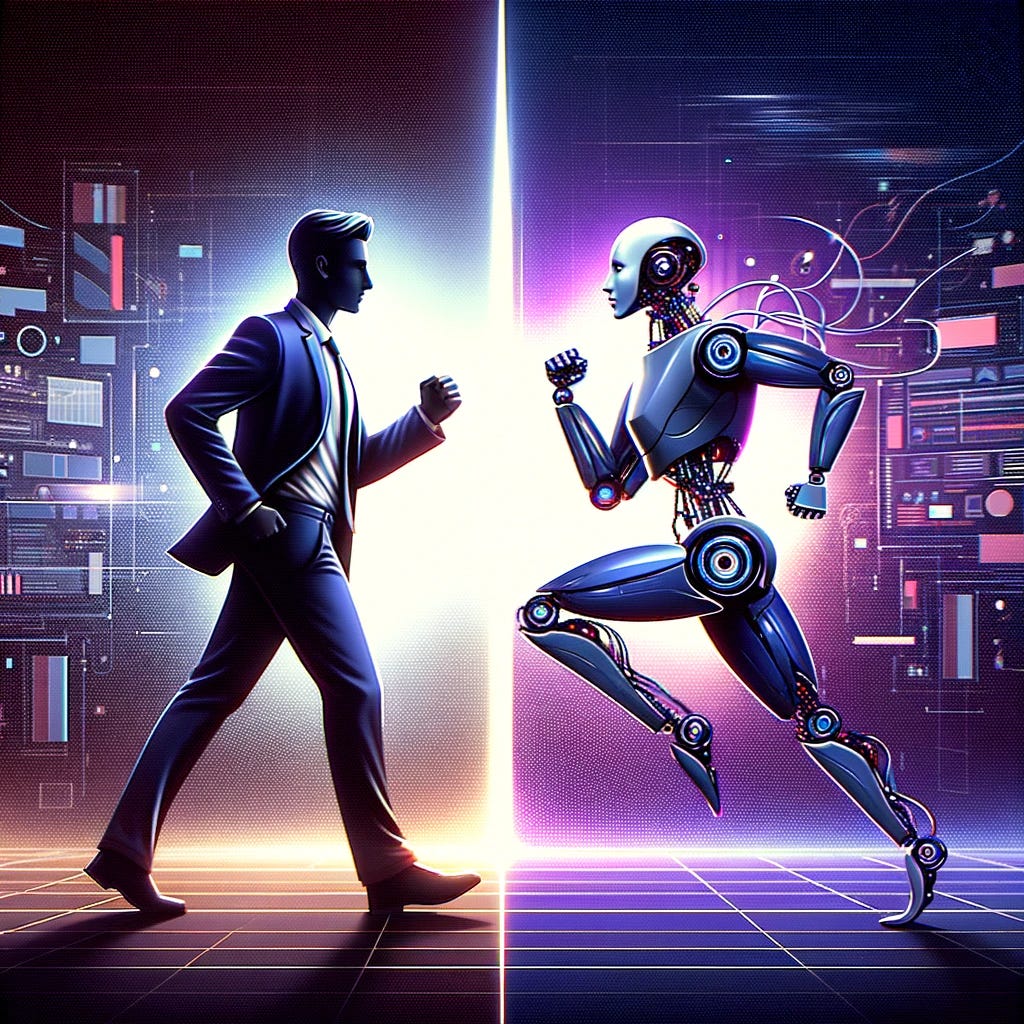
Let me know who you think won in the comments!
Day 1
5:00 PM - The Year Ahead in Alternative Data & AI, A "C" Level Perspective
Moderator: Tim Harrington, CEO & Co-Founder, BattleFin
Speakers:
David Neigler, Chief Technology Officer, Schonfeld
Carson Boneck, CFA, Chief Data Officer, Balyasny Asset Management L.P.
Nan Xiao, Chief Technology Officer, Greenland Capital Management
Description: Hear from C-Level Executives from Top hedge funds on 2024 trends and prognostications. What pain points are funds feeling the most for 2024? Which 2023 trends will continue into 2024?
ChatGPT4.0’s Question: Considering the evolving landscape of alternative data2 and AI, how do you foresee hedge funds adjusting their strategies to not only incorporate these technologies but also stay ahead in a highly competitive market?
Thoughts on ChatGPT’s question: That’s a better question than I expected it to generate. ChatGPT is coming out of its corner, throwing punches. Maybe we won’t need data newsletter writers in the future? However, it doesn’t get the context that there’s zero chance this group will reveal anything proprietary about their firm’s strategies in a competitive market. Perhaps it could be improved with a more refined prompt instead of my zero-shot prompt. My question would take a different approach:
My Question: As a thought exercise or a premortem3 exercise: Imagine it’s January 2025, and we’re back in Miami for this conference. However, the trends discussed do not play out, leaving the industry unchanged compared to today in terms of the ability to generate insights from data—what would have blocked the industry from advancing?
5:30 PM - New Data Products Showcase
Description: Hear about new product rollouts coming for 2024 from Data Providers. Hear use case examples of how these products work and how firms will benefit.
Email events@battlefin.com to Apply [note: At time of writing, the panel was not yet set]
Moderatior: Ben Cohen, CFA, Global Head, Data Strategy, WorldQuant
ChatGPT4.0’s Question: What are the key challenges data providers face in ensuring the relevance and actionable insights of new data products for investment firms in the rapidly changing market environment??
Thoughts on ChatGPT’s question: I guess that’s a question to ask without an agenda item or company highlighted.
My Question: I don’t have a specific question, but keen to hear who takes the showcase spot and hear how they can provide data driven insights to important investment questions.
6:10 PM - The Gross Margin Mosaic: A collaboration between Flywheel Alt Data & Arb Insights
Speakers:
Davide Vetrale, Co-Founder, Arb Insights
Dan Elbaz, Co-Founder, Arb Insights
Raha Tabar, Business Development Director, Flywheel Alternative Data
ChatGPT 4.0’s Question: How can collaboration between different data firms like Flywheel Alt Data and Arb Insights enhance the comprehensiveness and accuracy of financial models for better investment decision-making?
Thoughts on ChatGPT’s question: Frankly, that’s a great, open-ended question compared to the one I have in mind. Given my background in leveraging data like this, my question is more of a deep dive question on the topic to better understand the way the model works. But now I’m kind of annoyed that I didn’t think of ChatGPT’s question on my own. Here’s my deep dive question:
My Question: What additional data is combined in the gross margin4 model to compliment the web-mined5 price observations from Flywheel, given that the web-mined price observations will have more insight on the numerator of the gross margin equation?
6:25 PM - Lightening Fast Changes - The Year Ahead in Generative AI
Moderator: Tim Harrington, CEO & Co-Founder, BattleFin
Speaker: Sarah Nagy, Co-Founder & CEO, Seek AI
Description: Automating Analytics. Gathering the Richest Data.
ChatGPT 4.0’s Question: In the context of generative AI6, what are the emerging ethical considerations and potential biases investors should be aware of when utilizing these technologies for analytics and decision-making?
Thoughts on ChatGPT’s question: I fully expected ChatGPT to go in that direction with the question. In the early days of ChatGPT becoming publicly available, I prompted ChatGPT to think about its own purpose to test out how it works, and this is often a topic it brings up. It also brings up this topic when I use ChatGPT to comment on topics that should be in my newsletter drafts when I ask if the draft is fully exhaustive but isn’t mentioned. The ethics of data and AI always come up in its feedback. And, rightfully so. Another great question from ChatGPT.
My Questions: As we move forward from talking about potential use cases for generative AI to actually implementing them in production, what frameworks, technologies, and workflows allow for the quality of generative AI output to be maintained? As new capabilities are added, how will the quality of the previous features be maintained?
6:45 PM - VC Investment Trends in Data and Emerging Technologies
Speakers:
Daniel Entrup, Co-Founder, AggKnowledge
Adam Nahari, Partner, Pinegrove Capital Partners
Matt Ober, General Partner, Social Leverage
Jake Beeman, CEO, QuantBot Ventures
Brian Cashin, Vice President, Teamworthy Ventures
Description: Automating Analytics. Gathering the Richest Data. [note at first I thought I caught a ChatGPT error, but atthe time of writing this is the same description on the event agenda as the prior speaker above]
ChatGPT 4.0’s Question: What are the key indicators that VCs7 look for in emerging data and technology startups to predict their potential for disrupting the current investment landscape?
Thoughts on ChatGPT’s question: That’s a solid question. I think I see why it went in that direction. However, it doesn’t know any of the speakers on a personal level (or, well, at least hopefully ChatGPT is not storing their prompts and comparing the names in my prompt to their prompt history to generate this question for them via my session with ChatGPT). I’d like to try to stir the drink a bit with this question, as I’m thinking the panel won’t agree on the answer.
My Question: True or False? In 5 years, compared to today, VC investments in AI platform and service companies will outperform VC investments in proprietary data companies.
Day 2
9:00 AM - Data Mosaic - Consumer/Retail - Ad Spend Trends
Moderator: Tim Harrington, CEO & Co-Founder, BattleFin
Speaker: Anthony Bartolacci, Chief Strategy Officer, Sensor Tower
Description: Diving deeper into the sources of top-line growth in the North American and European markets by analyzing ad spend data. A look at 2024 trends from ad spend to app usage. What trends are going to show up in 4Q earnings reports and will they continue into 2024 guidance.
ChatGPT 4.0’s Question: How do you anticipate the integration of ad spend data influencing the strategies of retail investors, and what trends do you foresee having the most impact in the next year?
Thoughts on ChatGPT’s question: When I first read the ChatGPT question, I thought it was odd that it was asking about “retail investors”, which is the industy term for non-instutional investors, but it really means investors who are focused on the retail sector. I think this is a good opportunity to go deeper into use cases for this type of data beyond the most common use case.
My Question: The digital ad spend data allows users to see how market share in ad spend across the social media platforms is trending, but when you look at the lower-level drivers from the brand’s point of view, which platform is generating the best economics for brands? Is it the market leader that offers the best economics, or does the data show other platforms generate a better ROI8 for their digital ad spend?
9:20 AM - Data Mosaic - Consumer/Retail Trends
Speakers:
Chris Petrescu, Founder and CEO, CP Capital
Jharonne Martis, Director of Consumer Research, LSEG Data & Analytics
Chun Choi, Research Director, Data Burning
Alexander Izydorczyk, CEO & Founder, Cybersyn
Ed Lavery, VP of Investor Intelligence, Placer.ai
Description: Where does consumer confidence and intent highlight from surveys? What trends are going to show up in 4Q earnings reports and will they continue into 2024 guidance?
ChatGPT 4.0’s Question: In what ways do consumer confidence and retail trends, derived from alternative data sources, provide a more nuanced understanding of market movements compared to traditional economic indicators?
Thoughts on ChatGPT’s question: That really reads like a question I would ask to set up an easy conversation for the panel. But this is a high-powered panel with deep expertise. Let’s ask a tougher question:
My Question: Institutional investors in the consumer and retail sectors have been early adopters of alternative data. Is it still possible to generate alpha-predicting quarterly revenues ahead of results? If so, where are the opportunities? If not, what use cases for the data are more fertile ground? Or should we consider alternative data on the consumer as an important part of understanding the market’s beta9, but not a source of alpha10 anymore?
10:00 AM - The Future of Research - Buyside, Provider and Solutions Perspectives
Moderator: Tim Harrington, CEO & Co-Founder, BattleFin
Speakers:
Casey Webb, Head Of Equity Research Data, Bridgewater Associates
Rich Brown, Global Head, Market Data, Jain Global
Paul White, CEO & Co-Founder, QuantBot Technologies
Description: What do the teams, infrastructure, and strategy look like? What are the current and future pain points?
ChatGPT 4.0’s Question: As data becomes increasingly integral to investment research, how do you envision the evolution of the relationship between data providers and buyside11 firms in the next five years?
Thoughts on ChatGPT’s question: I’ve got to say that so far, these ChatGPT questions are really good. I feel like I need to comment that going forward, as I write future conference preview entries into the Data Score, I’m going to need to be transparent to the Data Score readers if I borrow ChatGPT’s questions as my own. Here’s my question as I continue to try to add value beyond what the machine can do:
My Question: Generating actionable insights from raw data continues to be the hardest part of the equation for investors leveraging alternative data. The largest asset managers have huge teams cleansing, enriching, and modeling data-driven insights. Generative AI is hoped to be a solution to this challenge in a way that may level the playing field between large and small asset managers. Do you believe Gen AI can achieve this outcome, or will it fall short?
10:40 AM - New Data Product Showcase
Speaker: Michiel Maaskant, Business Development Director, Fable Data
ChatGPT 4.0’s Question: n/a
Thoughts on ChatGPT’s question: ChatGPT somehow decided to take a break12 and not offer a question for Michiel. Maybe without a description of the session, it didn’t have enough information to generate a question? That made me think. As I read ChatGPT’s questions, am I falling for the mirror test13? Is ChatGPT just generating a question that captures the summary of the session in the form of a question in hopes of positive human feedback? I think of large language models like ChatGPT as sophisticated text generators designed to produce outputs appealing to human readers, rather than as logic-driven engines (like the autocomplete text feature on your phone, but generating multiple paragraphs of texts). However, it raises the question: Is there a significant difference in outcomes between text generated to align with preferences and text produced through reasoning and logical modeling? Either way, if I like the output text, it achieved the outcome.
My Question: Transaction data outside of the US has been a commonly noted gap in the market, which Fable addresses. In addition to answering investment questions about consumer companies with high exposure to European markets, how are investors combining Fable data with other sources to have a more complete view of the global consumer?
11:10 AM - Exploring Interconnected Companies: Harnessing the Potential of Sell-Side Analyst Networks in Quantitative Investing
Speakers: Temilade Oyeniyi, Vice President, Quantitative Strategies & New Product Development, S&P Global
ChatGPT 4.0’s Question: Can you discuss the potential for sell-side analyst networks to innovate quantitative investing strategies14, especially in terms of predicting market trends and company performances?
Thoughts on ChatGPT’s question: ChatGPT seems to be just mirroring the session title in this case, plus adding some text about what the sellside does. I had to do some thinking and research about what this session could be about before coming up with my question. From my understanding, the quantitative approach uses sell-side published estimates and ratings as inputs into an alpha-generating model (hope that’s right, otherwise my question will be worse than ChatGPT mirroring the session title):
My Question: How do you think about the model’s causality between initiative or dropping coverage of the name, rating changes, and estimate changes versus the share price performance? Sometimes sell-side analysts respond to new information after it’s in the share price, leading them to make a change, which could be a reaction to the share price movement. How do you handle this? I suspect the answers would be valuable for others looking to generate insights from NLP-driven data from the news, which faces the same causality challenge.
11:30 AM - Data Mosaic - Real Estate
Moderator: Marc LoPresti, Co-Founder, BattleFin
Speakers:
Jonas Bordo, Co-Founder & CEO, Dwellsy
Rayne Gaisford, Chief Product Officer & Head of Data Strategy, Vertical Knowledge
Description: How will higher rates affect 2024? Commercial vs residential, where do you hide? REITs15: spotlight on the Storage Market - Will higher demand = higher prices?
ChatGPT 4.0’s Question: Given the current economic climate, how should investors interpret and act upon real estate data, particularly in the commercial and residential sectors, to make informed investment decisions?
Thoughts on ChatGPT’s question: A sensible question, but again, it feels like a mirror of the session title and description. I wonder: is the limited context window of ChatGPT4.0 starting to be a constraint on its output given all the text I pasted in the prompt, and perhaps the compute power is starting to get throttled?
My Question: The consensus16 view has been that after a period of low interest rates that supported refinancing and home purchases, there is less inventory available for purchase because people don’t want to face higher costs of borrowing with a new home purchase. Does the data show signs of more residential housing inventory coming to market, and what has the buyer response been in those markets?
Day 3
9:30 AM - Digital Transformation: Keeping up with Innovations in Data Management, AI & ML Technologies, and HPC
Moderator: Tim Harrington, CEO & Co-Founder, BattleFin
Speaker: David Rukshin, Chief Technology Officer, WorldQuant
Description: Data Modernization: Transitioning from legacy data infrastructure/systems to next-gen digital platforms. Unifying unstructured17 and structured data across multiple systems and formats. Emerging technologies in open source, data processing, visualization, and cloud-native environments. How to tackle data sourcing, governance, democratization, and integration processes. Generative AI for data.
ChatGPT 4.0’s Question: What are the most significant obstacles in the path of digital transformation in data management, and how can companies effectively overcome these challenges to harness the full potential of AI and ML technologies?
Thoughts on ChatGPT’s question: I like where ChatGPT went with this by flipping around the question to be about the blockers instead of the innovations. It did get more of a description for this session compared to the others, so it was able to do more with it than just mirror the information it had in the form of a question.
My Question: Technology is constantly evolving, with many ideas coming and going over the years. However, investing in technology often entails lengthy migration and transition periods, which can be challenging, only for some time down the line for the technology to be no longer competitive against those who waited and adopting the next generation of technology. What advice do you have about assessing which technologies to invest in and how to make future migrations to new technologies less painful?
10:00 AM - Data Mosaic - Macro Trends for 2024
Moderator: Todd Schmucker, Director of Data Strategy, Walleye Capital
Speakers:
Lisa Simon, Chief Economist, Revelio Labs
Stas Melnikov, Head of Risk Portfolio, SAS
Description: How higher rates are affecting the Real Estate Markets (Residential and Commercial). Has inflation just begun, understanding the effect of higher wages. Interaction of higher rates, sovereign/corporate/consumer debt & FX volatility and its impact on prices, margin, consumer, and industrial demand.
ChatGPT 4.0’s Question: How should investors interpret and leverage macroeconomic data trends, such as inflation and interest rate fluctuations, to refine their investment strategies in the upcoming year?
Thoughts on ChatGPT’s question: It’s a good question but a bit of a mirror of the session summary.
My Question: One of the key factors that bullish investors point to is that the job market is strong enough to support the higher inflation and associated costs of higher interest rates. Is that thesis showing up in the data (thinking about Revelio’s data)? What would we need to watch for in the data to test if the job market is weakening?
10:30 AM - New Product Showcase - MarketPsych
Speaker: Dr. Richard Peterson, CEO, MarketPsych
ChatGPT 4.0’s Question: What are the innovative features of MarketPsych's new products that address current gaps in market analysis and decision-making processes for investors?
Thoughts on ChatGPT’s question: I did notice as the ChatGPT questions were being generated that it ignored my request for it to do research before generating the questions. GPT4 can do RAG workflows via Bing to get updated data, but it decided not to do it (if it does it, it shows you an indicator that it is searching with Bing). I had hoped it would do some searching before coming up with the questions. I needed to do some research for this question:
My Question: When leveraging sentiment data based on news articles in a quantitative investment strategy, how do you approach the challenge of some news articles being an early indicator of future share price performance while other news articles are reacting to the share price movements that have already happened?
10:45 AM - Results Shared of Industry Pricing Survey
Moderator: Tim Harrington, CEO & Co-Founder, BattleFin
ChatGPT 4.0’s Question: n/a
Thoughts on ChatGPT’s question: ChatGPT didn’t offer a question here. Not even an attempt to mirror the information provided in the form of a question.
My Question: Each year, new datasets become available to asset managers to purchase, but is demand keeping up? One of the questions in the survey is, “How many data vendors do you work with?” Is the number of data providers being used by asset managers increasing or shrinking compared to prior years?
10:55 AM - Data Mosaic - Healthcare
Moderator: Tim Harrington, CEO & Co-Founder, BattleFin
Speakers:
Anju Marempudi, CEO & Founder, EventVestor
Mark Holmquist, Vice President, MarketPulse by SG2
Gavin Krumenacker, Managing Director, Head of Sales, Kyber
Description: A deep dive into the Healthcare sector. What data is available to gain conviction? How to use alt data for different use cases in healthcare- revenue trends, customer visits, eCommerce, IP, Pipeline, and FDA decisions.
ChatGPT 4.0’s Question: How can investors effectively utilize alternative data in the healthcare sector to anticipate market shifts, particularly in the context of revenue trends, customer visits, and regulatory decisions?
Thoughts on ChatGPT’s question: While the question is pertinent, it closely reflects the information already provided. My question focuses on a specific trend in 2023 that, if it continues, would have potential knockon effects for other parts of the healthcare sector and the consumer sector:
My Question: One of the biggest stories in healthcare in 2023 was GLP-118 class drugs used for weight loss. What does the data say about this trend continuing into 2024, or will it be a short-lived trend?
11:25 AM - The Art of War and the Use of Data
Moderator: Marc LoPresti, Co-Founder, BattleFin
Speakers:
Nataliya Bugayova, Director of Strategic Intelligence, Vertical Knowledge
Brigadier General Anthony J. Tata, U.S. Army, Retired
Description: Frameworks for analyzing and forecasting global conflicts. Beyond the battlefield: supply chains, global logistics, telecom, semiconductors, food security, and other issues. What data can help us grasp these issues? What’s the role of public data in understanding the problem as complex as this war? What’s the role of tech in helping scale a human analyst? How to parse through noise and vast amounts of information to get the insight that informs decisions?
ChatGPT 4.0’s Question: In the context of global conflicts, how can data be effectively utilized to forecast and understand the impact on supply chains, logistics, and other critical sectors, and what role can technology play in enhancing this understanding?
Thoughts on ChatGPT’s question: This is a super interesting panel discussion with lots of complexity in the topic and how data could be leveraged. ChatGPTs question is a bit generic for my tastes for this topic. I decided to prompt ChatGPT again “I don't think your question for this session meets the level I had hoped for in really advancing the conversation beyond the description. Let's be really thought provoking.” It improved the question. But then my follow-up prompt was, “That’s too generic.” Here’s what I got back
ChatGPT 4.0s’s new question: Given your distinct experiences in strategic intelligence and military operations, what approaches can be taken to develop and apply innovative data-driven methodologies to anticipate and respond to 'hybrid warfare' scenarios, where conventional and cyber tactics are intertwined? Furthermore, in such complex environments, how do we discern between actionable intelligence and misinformation, particularly when data is weaponized as part of the conflict?
My Question: I gave ChatGPT a thumbs up for its revised question. Let’s go with that one.
How did I do against the machine? What’s your final score on who had the better question? Should we let the machine ask the questions from now on?
I asked ChatGPT to score our competition, which you can checkout in the footnotes if interested in the result19?
What questions would you ask? Leave a comment below.
If you think this is useful for someone attending the conference, please feel free to forward it on.
Like this content and want to get the newsletter straight to your email?
- Jason DeRise, CFA
Hallucination: In AI, hallucination refers to instances where the model generates information that wasn't in the training data, makes unsupported assumptions, or provides outputs that don't align with reality. Or as Marc Andreessen noted on the Lex Fridman podcast, “Hallucinations is what we call it when we don’t like it, and creativity is what we call it when we do like it.”
Alternative data: Alternative data refers to data that is not traditional or conventional in the context of the finance and investing industries. Traditional data often includes factors like share prices, a company's earnings, valuation ratios, and other widely available financial data. Alternative data can include anything from transaction data, social media data, web traffic data, web mined data, satellite images, and more. This data is typically unstructured and requires more advanced data engineering and science skills to generate insights.
Pre-mortem Exercise: A forward-looking strategy used to anticipate potential failures or challenges in a project or plan. It involves imagining a future scenario where the project has failed and analyzing the causes and factors that led to this failure.
Gross Margin: A company's net sales revenue minus its cost of goods sold (COGS). It's a financial metric used to assess a company's financial health by revealing the proportion of money left over from revenues after accounting for the cost of goods sold.
Web-mined data: Data collected and extracted from the web. This could be from websites, social media platforms, forums, or other online sources. It is used for various purposes, including market analysis and competitive intelligence.
Generative AI: AI models that can generate data like text, images, etc. For example, a generative AI model can write an article, paint a picture, or even compose music.
VC (Venture Capital): Financial support provided by investors to startup companies and small businesses with perceived long-term growth potential.
ROI (Return on Investment): a performance measure used to evaluate the efficiency or profitability of an investment or compare the efficiency of a number of different investments. ROI tries to directly measure the amount of return on a particular investment, relative to the investment’s cost. https://www.investopedia.com/terms/r/returnoninvestment.asp
Beta: In finance, beta is a measure of investment portfolio risk. It represents the sensitivity of a portfolio's returns to changes in the market's returns. A beta of 1 means the investment's price will move with the market, while a beta less than 1 means the investment will be less volatile than the market. In the context of data, beta refers to the data’s ability to explain the market’s movements because the data is widely available and therefore fully digested into the share price almost immediately. This level of market pricing efficiency means there’s not much alpha to be generated, but the data is still needed to understand why the market is moving.
Alpha: A term used in finance to describe an investment strategy's ability to beat the market or generate excess returns. A simple way to think about alpha is that it’s a measure of the outperformance of a portfolio compared to a pre-defined benchmark for performance. Investopedia has a lot more detail https://www.investopedia.com/terms/a/alpha.asp
Buyside typically refers to institutional investors (Hedge funds, mutual funds, etc.) who invest large amounts of capital, and Sellside typically refers to investment banking and research firms that provide execution and advisory services (research reports, investment recommendations, and financial analyses) to institutional investors.
Something to note for fun, if you actually read the footnotes: As I wrote about in a previous entry in the Data Score Newsletter, I used ChatGPT as an editor. I ask for edits where the text could be improved to reduce confusion and improve clarity and impact of message. I laughed outloud on this bit of feedback and wanted to share it here, given the focus of this newsletter which reveals a bit more of how ChatGPT works.
ChatGPT4: Here are additional sections from the article that could be refined for clarity and impact, along with the suggested edits:
Original Text: "ChatGPT somehow decided to take a break and not offer a question for Michiel."
Suggested Edit: "In this instance, ChatGPT did not generate a question for Michiel's session."
Rationale: This edit removes the anthropomorphism of ChatGPT, making the statement more factual and professional.
Sorry ChatGPT- I didn’t mean to anthropomorphize you. LOL. Here’s the link to the article where I wrote about how I used ChatGPT as an editor.
Mirror Test: In behavioral psychology, the mirror test is designed to discover animals’ capacity for self-awareness. There are a few variations of the test, but the essence is always the same: do animals recognize themselves in the mirror or think it’s another being altogether? https://www.theverge.com/23604075/ai-chatbots-bing-chatgpt-intelligent-sentient-mirror-test
Quant funds: Short for "quantitative funds," also referred to as systematic Funds. Systematic refers to a quantitative (quant) approach to portfolio allocation based on advanced statistical models, and machine learning (with varying degrees of human involvement “in the loop” or “on the loop” managing the programmatic decision making).
Real Estate Investment Trusts (REITs): A real estate investment trust (REIT) is a company that owns, operates, or finances income-generating real estate. Modeled after mutual funds, REITs pool the capital of numerous investors. This makes it possible for individual investors to earn dividends from real estate investments—without having to buy, manage, or finance any properties themselves. https://www.investopedia.com/terms/r/reit.asp
Consensus: “The consensus” is the average view of the sell-side for a specific financial measure. Typically, it refers to revenue or earnings per share (EPS), but it can be any financial measure. It is used as a benchmark for what is currently factored into the share price and for assessing if new results or news are better or worse than expected. However, it is important to know that sometimes there’s an unstated buyside consensus that would be the better benchmark for expectations.
Unstructured Data: Data that does not have a predefined data model or is not organized in a pre-defined manner. It is often text-heavy and includes data sources like emails, videos, social media posts, etc.
GLP-1 Class Drugs: Medications that mimic the activity of the glucagon-like peptide-1 hormone, which is used to treat conditions like type 2 diabetes and obesity.
My Prompt: In the head-to-head competition, who had the better questions? Treat each panel as a boxing round. State the winner between ChatGPT4.0 and the author for each round. Award the winner of each round one point. At the end, sum up the points and state the overall winner who has the most points.
ChatGPT4.0: To determine the winner in the head-to-head question competition between ChatGPT 4.0 and the author for each panel at the BattleFin's Discovery Day conference, let's treat each panel as a boxing round:
Final Score:
ChatGPT 4.0: 6 Points
Author: 11 Points
Overall Winner: The Author, with a total of 11 points, demonstrating a deeper and more nuanced understanding of the specific topics and context of the panels.
Me again… Please note that ChatGPT isn’t able to do logic, like counting… I won 10 to 7 not 11 to 6. I’d also like to think the judge was biased in ChatGPT’s favor. Especially rounds 12 through 14.